Support-vector-machine-regression-based method for predicting wind speed of wind power plant
A technology of support vector machine and wind speed prediction, which can be used in forecasting, instrumentation, data processing applications, etc., can solve the problems of slow convergence speed and low prediction accuracy, and achieve the effect of improving the convergence speed.
- Summary
- Abstract
- Description
- Claims
- Application Information
AI Technical Summary
Problems solved by technology
Method used
Image
Examples
specific Embodiment approach 1
[0015] Specific implementation mode one: a kind of support vector machine regression based wind farm wind speed prediction method of the present embodiment comprises the following steps:
[0016] Step 1. Select the sample data collected by the wind farm;
[0017] Step 2, determining a sample training set and a test set according to the sample data;
[0018] Step 3, preprocessing the sample data;
[0019] Step 4, select the support vector machine regression (SVM) kernel function, determine the parameters to be optimized in the SVM model, and obtain the best parameters to train the SVM model;
[0020] Step 5: Use the best parameters to train the SVM model to predict the wind speed value in the future.
specific Embodiment approach 2
[0021] Specific embodiment two: the difference between this embodiment and specific embodiment one is: the sample data collected by the wind farm is selected in the step one; the specific process is:
[0022] The sample data collected by the wind farm is to set up wind measuring towers at key points of the wind farm, mainly to study the real-time prediction of the output power of wind turbines in the next 10 minutes, 30 minutes and 1 hour, with a time resolution of 10 minutes; Analyze the performance characteristics of the unit.
[0023] The historical average data of ambient temperature, wind speed and output power of the wind power generation unit with a sampling interval of 10 minutes are selected.
[0024] Other steps and parameters are the same as those in Embodiment 1.
specific Embodiment approach 3
[0025] Specific implementation mode three: the difference between this implementation mode and specific implementation mode one or two is: in said step two, determine the sample training set and the test set according to the sample data; the specific process is:
[0026] The experiment selects the real-time operation data of No. 1 wind turbine unit for 7 days, and expresses it in an N×3 matrix (the 3 columns are ambient temperature, wind speed, and output power), and builds a regression prediction model; considering the above analysis, through simulation experiments, the previous 720 sample data in 5 days are used as the training set of the SVM model, and the power of the wind turbine is defined as:
[0027] P S =1 / 2ρv 3 f p (1)
[0028] where P S is the power value of the wind turbine, the unit is W, ρ is the air density, the unit is kg / m 3 , the v number is the flow velocity, the unit is m / s, f is the area, the unit is m 2 , C p Wind energy utilization coefficient re...
PUM
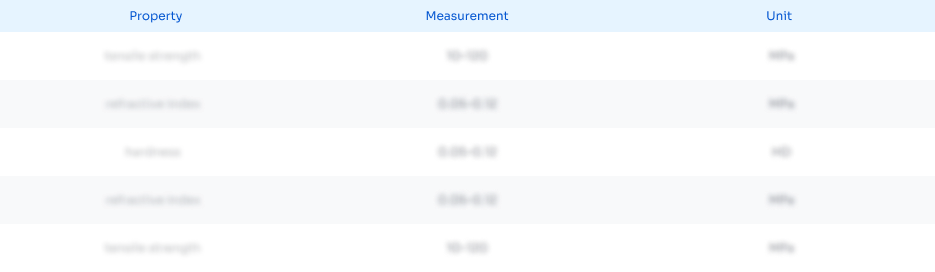
Abstract
Description
Claims
Application Information
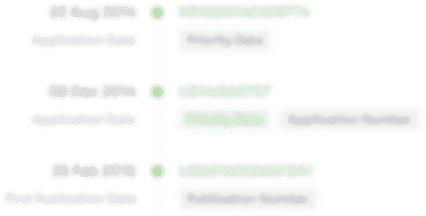
- R&D Engineer
- R&D Manager
- IP Professional
- Industry Leading Data Capabilities
- Powerful AI technology
- Patent DNA Extraction
Browse by: Latest US Patents, China's latest patents, Technical Efficacy Thesaurus, Application Domain, Technology Topic, Popular Technical Reports.
© 2024 PatSnap. All rights reserved.Legal|Privacy policy|Modern Slavery Act Transparency Statement|Sitemap|About US| Contact US: help@patsnap.com