Video abnormity detection method based on graph structure under multi-scale transformation
An anomaly detection and graph structure technology, which is applied in the direction of instruments, character and pattern recognition, computer components, etc., can solve the problem of high time-consuming impact on anomaly detection, fast detection, cumbersome implementation of mixed dynamic texture features, and inability to realize anomaly detection in real time and other issues, to achieve the effect of improving computational efficiency, increasing computational efficiency, and reducing the number of optical flow features
- Summary
- Abstract
- Description
- Claims
- Application Information
AI Technical Summary
Problems solved by technology
Method used
Image
Examples
Embodiment Construction
[0042] The implementation flow chart of the present invention is as figure 1 As shown, the specific implementation steps are as follows:
[0043] Step 1: Read in the UMN public video data set with a resolution of 240*320, the video images of the training set and the test set, set the grid size to 24*32, select a 10*10 pixel unit to extract its displacement information, and use the pyramid L-K The optical flow algorithm calculates the motion optical flow features (u, v) of each frame. Among them, u and v are the size of the horizontal velocity field and the size of the vertical velocity field of the movement of the points on the two adjacent frames of grids.
[0044] Step 2: Select one of the optical flow features to compare with the set threshold T, color code the optical flow components higher than the threshold T, and accumulate and record the change area of the color block to obtain the boundary contour of the motion area.
[0045] The threshold T is 0.095.
[0046] St...
PUM
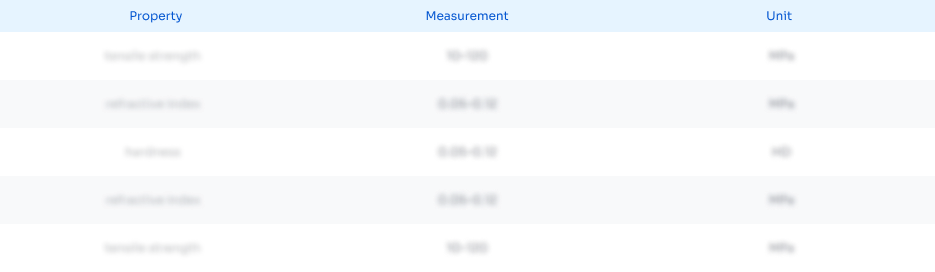
Abstract
Description
Claims
Application Information
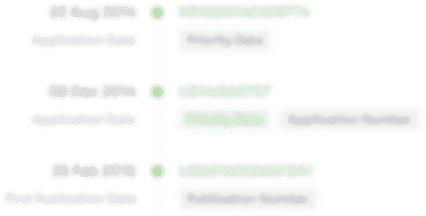
- R&D Engineer
- R&D Manager
- IP Professional
- Industry Leading Data Capabilities
- Powerful AI technology
- Patent DNA Extraction
Browse by: Latest US Patents, China's latest patents, Technical Efficacy Thesaurus, Application Domain, Technology Topic, Popular Technical Reports.
© 2024 PatSnap. All rights reserved.Legal|Privacy policy|Modern Slavery Act Transparency Statement|Sitemap|About US| Contact US: help@patsnap.com