DEM super-resolution method based on convolutional neural network
A convolutional neural network and super-resolution technology, applied in the field of terrain mapping, can solve the problems of similarity-dependent determination and small adaptability, and achieve the effects of high accuracy, improved accuracy and improved robustness
- Summary
- Abstract
- Description
- Claims
- Application Information
AI Technical Summary
Problems solved by technology
Method used
Image
Examples
Embodiment 1
[0065] This embodiment 1 includes the following steps:
[0066] (1) Use the bicubic interpolation method to expand the low-resolution DEM data by s times to obtain the quasi-high-resolution DEM data of the same scale as the expected high-resolution DEM data.
[0067] (2) Use Sobel operator to extract edge maps in X and Y directions of quasi-high resolution DEM data.
[0068] (3) Input the gradient map into the super-resolution convolutional neural network trained on the high- and low-resolution image data to obtain the estimated gradient map of the high-resolution DEM data;
[0069] Wherein, the super-resolution convolutional neural network trained on the high and low resolution image data is obtained according to the following steps:
[0070] (3-1) Acquire a large amount of image high-resolution data, perform double-triple down-sampling and then double-triple up-sampling on all images in the image database to obtain the corresponding low-resolution image data, and use the high- and low...
PUM
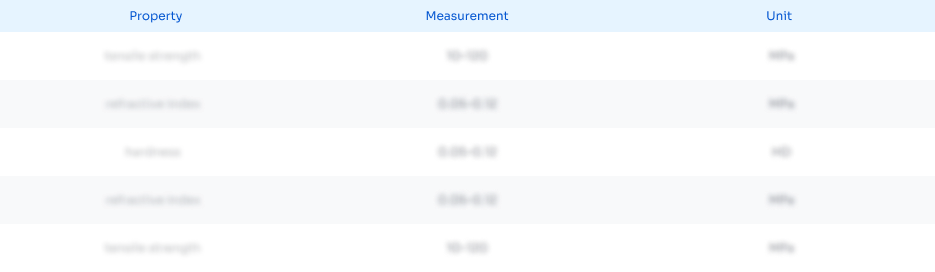
Abstract
Description
Claims
Application Information
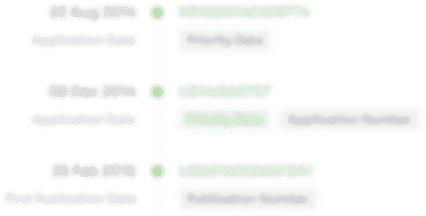
- R&D
- Intellectual Property
- Life Sciences
- Materials
- Tech Scout
- Unparalleled Data Quality
- Higher Quality Content
- 60% Fewer Hallucinations
Browse by: Latest US Patents, China's latest patents, Technical Efficacy Thesaurus, Application Domain, Technology Topic, Popular Technical Reports.
© 2025 PatSnap. All rights reserved.Legal|Privacy policy|Modern Slavery Act Transparency Statement|Sitemap|About US| Contact US: help@patsnap.com