An Integrated Semi-Supervised Fisher Discriminant Based Fault Classification Method for Industrial Processes
A Fisher discrimination and fault classification technology, applied in character and pattern recognition, database models, instruments, etc., can solve the problems of unstable performance of semi-supervised learning, not as good as supervised learning, etc., which is conducive to automatic implementation and improved monitoring. Effects, effects that enhance the mastery of the state of the process
- Summary
- Abstract
- Description
- Claims
- Application Information
AI Technical Summary
Problems solved by technology
Method used
Image
Examples
Embodiment Construction
[0018] The present invention is aimed at the problem of fault classification in industrial processes. In this method, firstly, the distributed control system is used to collect data under normal working conditions and several kinds of fault data as training data sets. First, offline modeling is performed, and a large amount of unlabeled data is randomly sampled. , and form several random training subsets with all the labeled data, and then perform semi-supervised Fisher dimensionality reduction to obtain multiple Fisher discriminant matrices (composed of r Fisher discriminant vectors, r is dimensionality reduction dimension). Bayesian classification is performed on the sample data after dimensionality reduction to obtain a series of posterior probability matrices, and the posterior probability matrices of labeled data and the corresponding labels are used as training samples for the K-nearest neighbors of the metric layer fusion algorithm. Finally, during online classification...
PUM
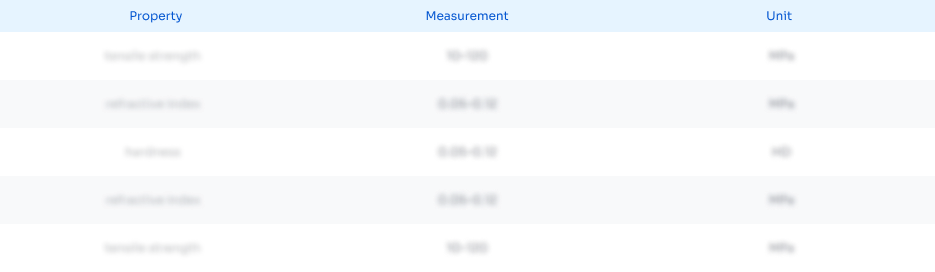
Abstract
Description
Claims
Application Information
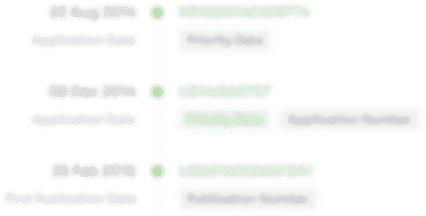
- R&D Engineer
- R&D Manager
- IP Professional
- Industry Leading Data Capabilities
- Powerful AI technology
- Patent DNA Extraction
Browse by: Latest US Patents, China's latest patents, Technical Efficacy Thesaurus, Application Domain, Technology Topic.
© 2024 PatSnap. All rights reserved.Legal|Privacy policy|Modern Slavery Act Transparency Statement|Sitemap