Data flow abnormality detection method based on parallel Kalman algorithm
A technology of anomaly detection and data flow, applied in the field of big data management, which can solve problems such as inaccuracy and efficiency of anomaly detection
- Summary
- Abstract
- Description
- Claims
- Application Information
AI Technical Summary
Problems solved by technology
Method used
Image
Examples
Embodiment Construction
[0061] Below in conjunction with specific embodiment, further illustrate the present invention, should be understood that these embodiments are only used to illustrate the present invention and are not intended to limit the scope of the present invention, after having read the present invention, those skilled in the art will understand various equivalent forms of the present invention All modifications fall within the scope defined by the appended claims of the present application.
[0062] The parallel Kalman algorithm based on multi-dimensional impact factors, first of all, in order to improve the accuracy of abnormal detection results, and to determine the type and cause of abnormality, the anomaly detection of data streams adds three dimensions of time, space and other origin information. Influencing factors, a Kalman method based on multi-dimensional influencing factors is proposed; then, in order to improve the efficiency of the algorithm, the algorithm is decomposed into...
PUM
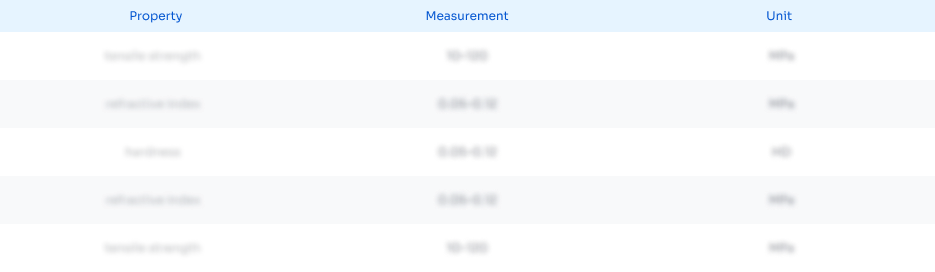
Abstract
Description
Claims
Application Information
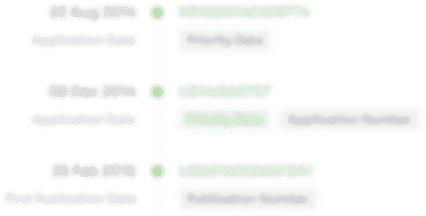
- R&D Engineer
- R&D Manager
- IP Professional
- Industry Leading Data Capabilities
- Powerful AI technology
- Patent DNA Extraction
Browse by: Latest US Patents, China's latest patents, Technical Efficacy Thesaurus, Application Domain, Technology Topic.
© 2024 PatSnap. All rights reserved.Legal|Privacy policy|Modern Slavery Act Transparency Statement|Sitemap