A method for end-to-end robotic arm control based on deep learning
A technology of deep learning and manipulators, applied in manipulators, program-controlled manipulators, manufacturing tools, etc., can solve the problems of high data cost and difficult data acquisition
- Summary
- Abstract
- Description
- Claims
- Application Information
AI Technical Summary
Problems solved by technology
Method used
Image
Examples
Embodiment Construction
[0022] The invention discloses an end-to-end robotic arm control method based on deep learning,
[0023] Step 1, collecting image information and control instructions and state information of the robotic arm at a certain frequency when the robotic arm is in motion;
[0024] Step 2, performing network processing on the image information, control instructions and status information collected in step 1:
[0025] The network includes an image processing network and a control strategy network. The image processing network is used to reduce the dimensionality of the original image, so that the image input and the state input dimension of the manipulator are on the same order of magnitude;
[0026] The control strategy network outputs the control instructions executed on the robotic arm, initializes the weight of the image processing network, the image information is used as input, and the status information of the robotic arm is used as output, training and initializing the image pr...
PUM
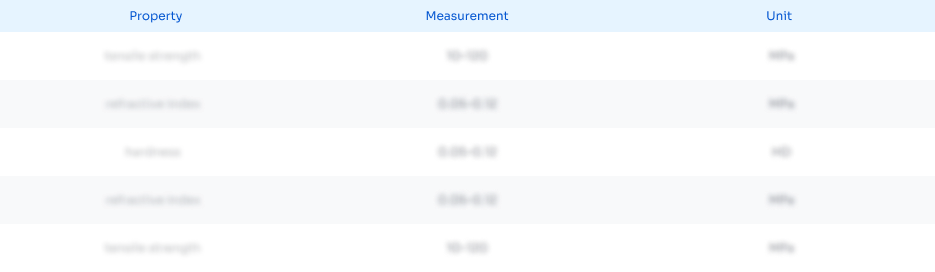
Abstract
Description
Claims
Application Information
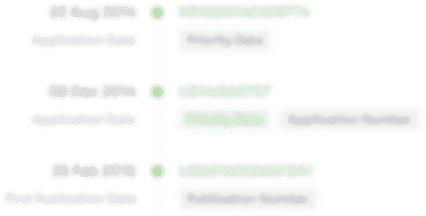
- R&D Engineer
- R&D Manager
- IP Professional
- Industry Leading Data Capabilities
- Powerful AI technology
- Patent DNA Extraction
Browse by: Latest US Patents, China's latest patents, Technical Efficacy Thesaurus, Application Domain, Technology Topic, Popular Technical Reports.
© 2024 PatSnap. All rights reserved.Legal|Privacy policy|Modern Slavery Act Transparency Statement|Sitemap|About US| Contact US: help@patsnap.com