A Generalization Algorithm for Manifold Learning Based on Local Linear Regression
A manifold learning, local linear technology, applied in computing, computer components, instruments, etc., can solve the problems of inability to directly learn hyperspectral data and lack of generalization ability.
- Summary
- Abstract
- Description
- Claims
- Application Information
AI Technical Summary
Problems solved by technology
Method used
Image
Examples
Embodiment Construction
[0039] The technical solution of the present invention will be further described below in conjunction with the accompanying drawings, but it is not limited to this. Any modification or equivalent replacement of the technical solution of the present invention without departing from the spirit and scope of the technical solution of the present invention should be covered by the technical solution of the present invention. in the scope of protection.
[0040] The hyperspectral image data used in the experiment uses the IND PINE hyperspectral data to conduct simulation research on the algorithm proposed by the present invention. This hyperspectral image was taken by the Kennedy Space Center in the United States on a farmland in Indiana, USA. There are 16 different crops in this image, and the spatial resolution of the image is 20×20m 2 , each pixel has 224 bands, covering the spectral range of 0.2-2.4 μm, and the spectral resolution is 10nm.
[0041] Randomly extract 1000 samples...
PUM
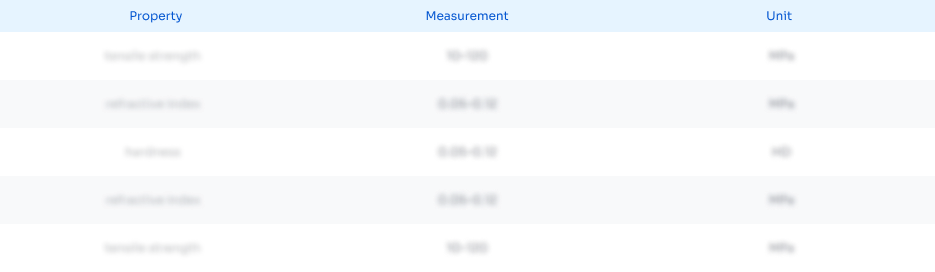
Abstract
Description
Claims
Application Information
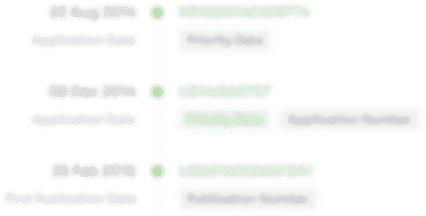
- R&D Engineer
- R&D Manager
- IP Professional
- Industry Leading Data Capabilities
- Powerful AI technology
- Patent DNA Extraction
Browse by: Latest US Patents, China's latest patents, Technical Efficacy Thesaurus, Application Domain, Technology Topic, Popular Technical Reports.
© 2024 PatSnap. All rights reserved.Legal|Privacy policy|Modern Slavery Act Transparency Statement|Sitemap|About US| Contact US: help@patsnap.com