Double-frame depth and motion estimating method based on convolutional neural network
A convolutional neural network, dual-frame technology, applied in biological neural network models, neural architectures, computing, etc., can solve problems such as limited application scope, inaccurate depth and camera motion estimation, and achieve the effect of saving memory
- Summary
- Abstract
- Description
- Claims
- Application Information
AI Technical Summary
Problems solved by technology
Method used
Image
Examples
Embodiment Construction
[0027] It should be noted that, in the case of no conflict, the embodiments in the present application and the features in the embodiments can be combined with each other. The present invention will be further described in detail below in conjunction with the drawings and specific embodiments.
[0028] figure 1 It is a system flowchart of a method for estimating depth and motion of a dual-frame based convolutional neural network in the present invention. It mainly includes image input; self-service network processing; iterative processing; image refinement; and obtaining estimation results.
[0029] Wherein, in the image input, an indoor scene image with depth and camera pose is selected as a scene data set, including a variety of different scenes from cartoon to realistic; when sampling image pairs from the data set, automatically discard those with highlight consistency image pairs, and split the dataset such that the same scene does not appear in both the training and test...
PUM
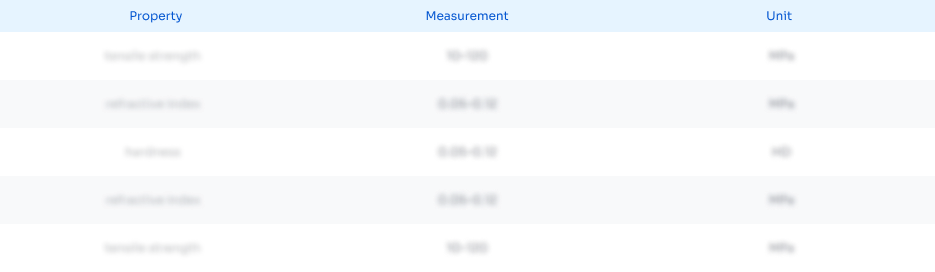
Abstract
Description
Claims
Application Information
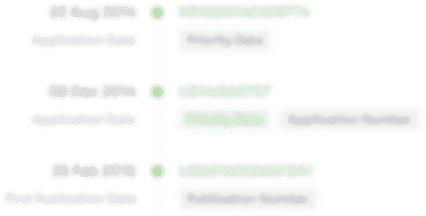
- R&D Engineer
- R&D Manager
- IP Professional
- Industry Leading Data Capabilities
- Powerful AI technology
- Patent DNA Extraction
Browse by: Latest US Patents, China's latest patents, Technical Efficacy Thesaurus, Application Domain, Technology Topic, Popular Technical Reports.
© 2024 PatSnap. All rights reserved.Legal|Privacy policy|Modern Slavery Act Transparency Statement|Sitemap|About US| Contact US: help@patsnap.com