Speech recognition method adopting rectified deep auto-encoder network
A technology of self-encoding network and speech recognition, which is applied in the field of corrected linear deep self-encoding network speech recognition, to achieve the effect of optimizing model training algorithm, improving training efficiency, enhancing recognition performance and anti-noise ability
- Summary
- Abstract
- Description
- Claims
- Application Information
AI Technical Summary
Problems solved by technology
Method used
Image
Examples
Embodiment Construction
[0033] The specific embodiment of the present invention is further described as follows.
[0034] Since autoencoders can be quickly trained by an unsupervised layer-by-layer greedy training algorithm, this approach bypasses the high complexity of directly training a DAE as a whole and reduces it to training multiple autoencoders question. After training in this way, the network is fine-tuned through the traditional global learning algorithm, and the maximum likelihood function is used as the objective function to make the network optimal. This learning algorithm is essentially equivalent to pre-training the layer-by-layer autoencoder to obtain a better initial parameter value for the model, and then further training and optimizing the network by using a small number of traditional learning algorithms.
[0035] During training, for the DAE model with the traditional Sigmoid function as the activation function, when using the backpropagation algorithm to transfer the error from...
PUM
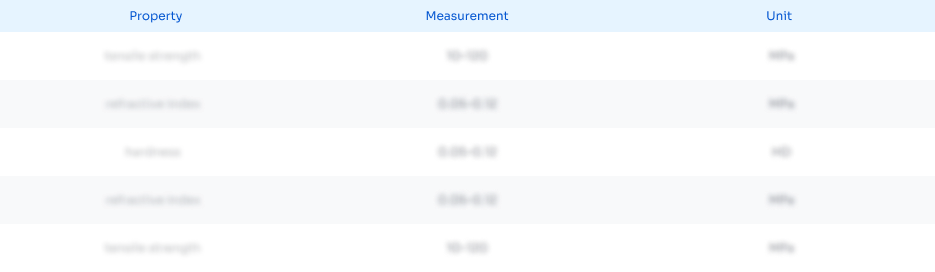
Abstract
Description
Claims
Application Information
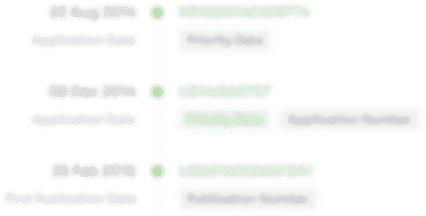
- R&D Engineer
- R&D Manager
- IP Professional
- Industry Leading Data Capabilities
- Powerful AI technology
- Patent DNA Extraction
Browse by: Latest US Patents, China's latest patents, Technical Efficacy Thesaurus, Application Domain, Technology Topic.
© 2024 PatSnap. All rights reserved.Legal|Privacy policy|Modern Slavery Act Transparency Statement|Sitemap