Method of identifying and classifying transient electric energy quality recording data
A technology of transient power quality and recorded wave data, applied in character and pattern recognition, instruments, computer parts, etc., can solve the problems of complex and huge input data, slow training speed, and difficulty in neural network recognition.
- Summary
- Abstract
- Description
- Claims
- Application Information
AI Technical Summary
Problems solved by technology
Method used
Image
Examples
Embodiment 1
[0031] The identification and classification method of transient power quality recording data provided by the present invention comprises the following steps:
[0032] Step S110, acquiring power quality wave data x(t), and filtering training data and test data from the data x(t). Specifically, such as image 3 As shown, step S110 also includes the following steps:
[0033] Step S111, collect and acquire real-time power quality wave data on the user side or the system side in real time. The real-time power quality wave data is the voltage amplitude variation curve at different times, which is the power quality wave data x(t).
[0034] Step S112, randomly sampling in the real-time power quality recording data, and selecting training data for training the subsequent BP neural network. Step S113, using the real-time power quality recording data as test data to detect the user's power quality condition.
[0035] Step S120, transforming the data into a modulo-time-frequency matri...
Embodiment 2
[0066] In step S110, in addition to the implementation mode provided in Example 1, as Figure 4 As shown, the following steps may also be specifically included:
[0067] Step S114, collect and obtain the real-time power quality wave data of the power quality on the user side or the system side in real time, and at the same time collect the historical power quality wave data stored in the database, the real-time power quality wave data and the historical power quality wave data. The wave data are amplitude change curves at different times, and they are all wave data of power quality recording.
[0068] Step S115, randomly sampling in the real-time power quality recording data, and selecting the training data for training the subsequent BP neural network; or randomly sampling in the real-time power quality recording data and historical power quality recording data, and selecting training data for the subsequent BP neural network The training data for training the network; or ra...
Embodiment 3
[0071] In step S110, in addition to the implementations provided in Embodiment 1 and Embodiment 2, as Figure 5 As shown, the following steps may also be specifically included:
[0072]Step S117, generating simulation data based on MATLAB software simulation, wherein the simulation data includes voltage sag data, voltage swell data, voltage interruption data, transient oscillation data and transient pulse data. Specifically, the expression of the simulation data of power quality is shown in Table 1.
[0073] Table 1 Expression of simulation data of power quality
[0074]
[0075] Step S118, obtaining simulation data and real-time power quality recording data.
[0076] In step S119, the simulation data is used as training data, and the real-time power quality recording data is used as test data.
[0077] At this time, since the type of simulation data is known, there is no need to judge and classify the type of simulation data through the historical data characteristics o...
PUM
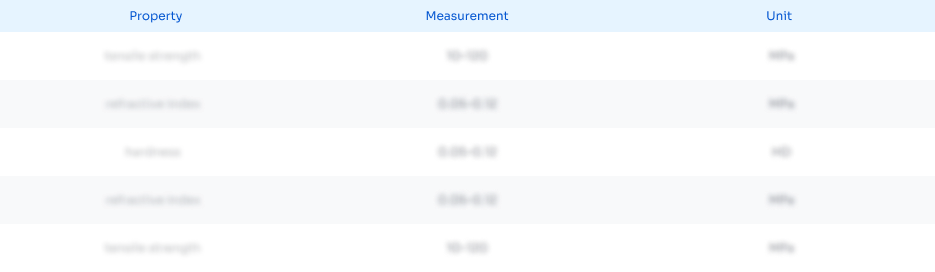
Abstract
Description
Claims
Application Information
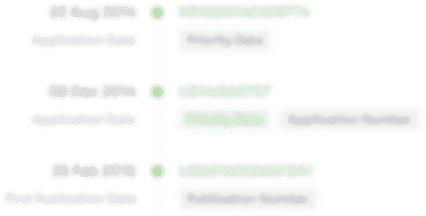
- R&D Engineer
- R&D Manager
- IP Professional
- Industry Leading Data Capabilities
- Powerful AI technology
- Patent DNA Extraction
Browse by: Latest US Patents, China's latest patents, Technical Efficacy Thesaurus, Application Domain, Technology Topic, Popular Technical Reports.
© 2024 PatSnap. All rights reserved.Legal|Privacy policy|Modern Slavery Act Transparency Statement|Sitemap|About US| Contact US: help@patsnap.com