Gradient descent and generalized inverse-based complex-valued neural network training method
A technology of neural network training and gradient descent method, which is applied in the field of complex-valued neural network training, can solve the problems of low precision and slow training speed, and achieve the effect of high precision, small number and few iterations
- Summary
- Abstract
- Description
- Claims
- Application Information
AI Technical Summary
Problems solved by technology
Method used
Image
Examples
Embodiment 1
[0060] In this embodiment, the three-dimensional equalizer model of the nonlinear distortion of the 4-QAM signal in the document "Channel Equalization Using Adaptive Complex Radial Basis Function Networks" is cited. Among them, the input of the equalizer is
[0061] The ideal output of the equalizer is 0.7+0.7i, 0.7-0.7i, -0.7+0.7i, -0.7-0.7i.
[0062] In this embodiment, the training data set and the testing data set take 70% and 30% of the overall sample data set respectively.
[0063] First, the data set is modeled by a complex-valued neural network training method based on the gradient descent method and the generalized inverse of the present invention.
[0064] A complex-valued neural network training method based on gradient descent method and generalized inverse, the method flow chart is as follows figure 1 As shown, the method steps include:
[0065] (1) Select a single hidden layer complex-valued neural network model to model the sample training data set or the s...
PUM
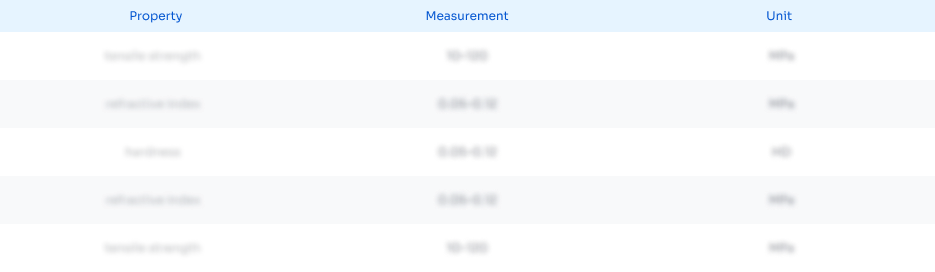
Abstract
Description
Claims
Application Information
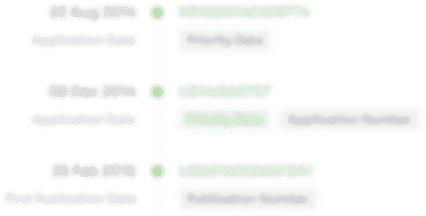
- R&D
- Intellectual Property
- Life Sciences
- Materials
- Tech Scout
- Unparalleled Data Quality
- Higher Quality Content
- 60% Fewer Hallucinations
Browse by: Latest US Patents, China's latest patents, Technical Efficacy Thesaurus, Application Domain, Technology Topic, Popular Technical Reports.
© 2025 PatSnap. All rights reserved.Legal|Privacy policy|Modern Slavery Act Transparency Statement|Sitemap|About US| Contact US: help@patsnap.com