Feedforward sequence memory neuron network and construction method and system thereof
A neural network and memory technology, applied in the field of artificial intelligence, can solve problems such as the inability of neural networks to guarantee information processing efficiency.
- Summary
- Abstract
- Description
- Claims
- Application Information
AI Technical Summary
Problems solved by technology
Method used
Image
Examples
Embodiment 1
[0072] A kind of feed-forward sequence memory neural network comprises a plurality of nodes of at least three layers, the first layer is an input layer, the last layer is an output layer, and other multiple nodes located between the input layer and the output layer form at least one hidden layer, The nodes between the layers are fully connected, and also include: each hidden layer contains a memory block, and the hidden layer and the memory block together constitute the FSMN layer of the bidirectional feed-forward sequence memory neural network, where the memory block of the current hidden layer The input of is the output of the current hidden layer, and the output of the memory block of the current hidden layer is an input of the next hidden layer. The memory block is used to store the historical information and future information of the input data of each frame, and the historical information is the current frame The feature sequence of the frame before the input data, the fu...
Embodiment 2
[0075] A feedforward sequence memory neural network, as described in Embodiment 1, the difference is that in this embodiment, in order to improve the ability of the neural network to process information data, the bidirectional FSMN stack is replaced by a bidirectional LSFSMN stack, Each bidirectional LSFSMN layer is composed of a bidirectional FSMN layer and an LSTM layer of the same layer, wherein the LSTM layer is used to store historical information, and the bidirectional FSMN layer is used to store future information. The memory capability of the neural network structure for input data is better than that of the neural network structure provided in Embodiment 1.
[0076] A kind of feed-forward sequence memory neural network comprises a plurality of nodes of at least three layers, the first layer is an input layer, the last layer is an output layer, and other multiple nodes located between the input layer and the output layer form at least one hidden layer, The nodes betwee...
Embodiment 3
[0078] A feed-forward sequence memory neural network is as described in the first embodiment, the difference is that in this embodiment, in order to improve the ability of the neural network to process information data, the neural network structure also includes a fully connected stack. The information processing capability of the neural network structure is better than that of the neural network structure provided in Embodiment 1, and the information processing efficiency will not decrease significantly.
[0079] A kind of feed-forward sequence memory neural network comprises a plurality of nodes of at least three layers, the first layer is an input layer, the last layer is an output layer, and other multiple nodes located between the input layer and the output layer form at least one hidden layer, The nodes between layers are fully connected, each hidden layer contains a memory block, and the hidden layer and the memory block together constitute the FSMN layer of the bidirect...
PUM
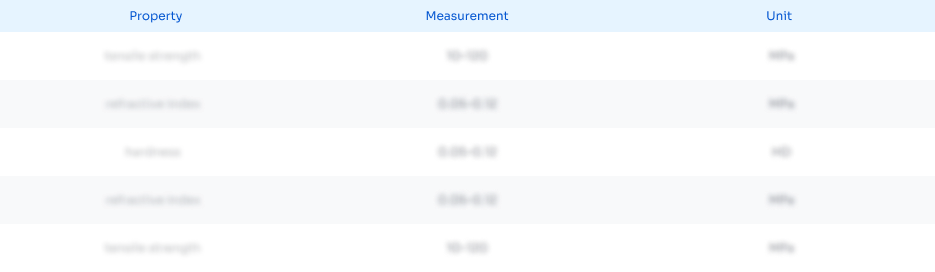
Abstract
Description
Claims
Application Information
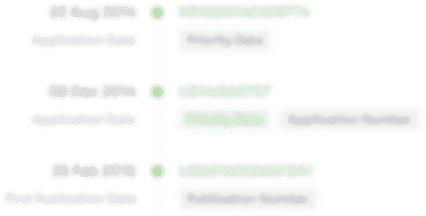
- R&D Engineer
- R&D Manager
- IP Professional
- Industry Leading Data Capabilities
- Powerful AI technology
- Patent DNA Extraction
Browse by: Latest US Patents, China's latest patents, Technical Efficacy Thesaurus, Application Domain, Technology Topic, Popular Technical Reports.
© 2024 PatSnap. All rights reserved.Legal|Privacy policy|Modern Slavery Act Transparency Statement|Sitemap|About US| Contact US: help@patsnap.com