Human body-behind-wall multi-state target detection method based on fuzzy pattern recognition and genetic algorithm
A technology of fuzzy pattern recognition and genetic algorithm, applied in the field of fuzzy pattern recognition and genetic algorithm detection technology, can solve the problems of not much research, unsuitable layout, high cost and unsuitable portability, etc., to achieve the effect of accurate target recognition algorithm
- Summary
- Abstract
- Description
- Claims
- Application Information
AI Technical Summary
Problems solved by technology
Method used
Image
Examples
Embodiment 1
[0046] The basic idea of human body target recognition behind the wall is to process the received signal of UWB radar equipment, and extract the characteristic parameters related to the target information from the received signal. The present invention mainly extracts the characteristic parameters of the received signal, and extracts six characteristics for each state. Parameters, including Kurtosis, Skweness, the maximum amplitude of the received signal, the variance and covariance of the received signal. The Gaussian function is selected as the sub-membership function, and each feature parameter of each scene corresponds to a sub-membership function. There are 36 sub-membership functions in 6 scenes, and the mean and variance of the sub-membership functions are obtained by genetic algorithm. The membership function set of fuzzy pattern recognition is constructed through the sub-membership function, and the state of the human target behind the wall is classified and recogniz...
Embodiment 2
[0053] We selected 6 states behind the wall for experiments, namely the state of no one behind the wall, the state of 1 person behind the wall breathing slowly, the state of 1 person waving his arms behind the wall, the state of 2 people behind the wall breathing slowly, and the state of 2 people walking back and forth behind the wall , the 3 people behind the wall breathe slowly. We choose 500 sets of pulse data for each state, and we choose 1152 data points for each set of pulse data. from figure 1 You can see the time-domain waveform diagram of each state, and the difference between various states can be seen weakly from the amplitude of the fluctuation. Therefore, the characteristic parameters are extracted for each state, and the target is identified through the characteristic parameters.
Embodiment 3
[0055] Extract the characteristic parameters of the received signal in each state, which are kurtosis, skewness, maximum amplitude of the received signal, energy, variance and covariance of the received signal. The sub-membership function is constructed through the characteristic parameters, and then the membership function set is formed. The Gaussian function is used as a sub-membership function, and its mean and variance are obtained by genetic algorithm. figure 2 It represents the mean value and variance corresponding to the 6 characteristic parameters of the 6 states behind the wall. Through the target prediction function and the principle of maximum membership degree of fuzzy pattern recognition, the test data is brought into the target prediction function to classify and judge the data category. . from image 3 Among them, the recognition effect of various states behind the wall can be clearly seen through the ROC curve.
PUM
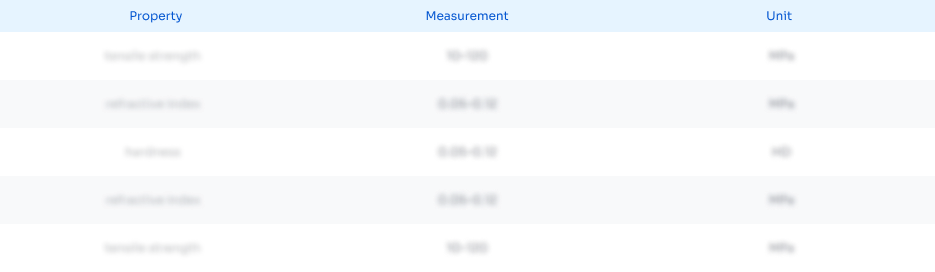
Abstract
Description
Claims
Application Information
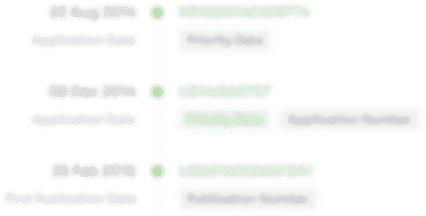
- R&D Engineer
- R&D Manager
- IP Professional
- Industry Leading Data Capabilities
- Powerful AI technology
- Patent DNA Extraction
Browse by: Latest US Patents, China's latest patents, Technical Efficacy Thesaurus, Application Domain, Technology Topic, Popular Technical Reports.
© 2024 PatSnap. All rights reserved.Legal|Privacy policy|Modern Slavery Act Transparency Statement|Sitemap|About US| Contact US: help@patsnap.com