Monocular vision-based lane line and front obstacle detection method
An obstacle detection and monocular vision technology, applied in the field of image processing, can solve the problems of inaccurate detection of lane lines and low detection accuracy of obstacles ahead, and achieve the goals of reducing the total amount of calculation, improving driving safety, and improving recognition accuracy Effect
- Summary
- Abstract
- Description
- Claims
- Application Information
AI Technical Summary
Problems solved by technology
Method used
Image
Examples
specific Embodiment approach 1
[0045] Specific implementation mode one: combine figure 1 To illustrate this embodiment, the specific process of a method for detecting lane lines and obstacles ahead based on monocular vision in this embodiment is as follows:
[0046] Step 1, using a vehicle-mounted CCD camera to obtain an original image, and performing grayscale processing on the original image to obtain a grayscale image;
[0047] Step 2, preprocessing the grayscale image to obtain a binarized image after removing clutter;
[0048] Step 3. Carry out the initial detection of the lane line in the near half area and the detection of the line pressure alarm based on the Hough transform on the binarized image after the clutter is removed;
[0049] Step 4, according to the point coordinates on the initial detection line of the lane line in the near half area obtained in step 3, carry out parabola fitting to the lane line in the near field of vision and the lane line in the far field of view, to obtain the fitte...
specific Embodiment approach 2
[0051] Specific embodiment 2: The difference between this embodiment and specific embodiment 1 is that the grayscale image is preprocessed in the step 2; the preprocessing includes binarization and filtering of the grayscale image; the binary value after removing clutter is obtained Image; the specific process is:
[0052] Step 21. Perform binary preprocessing on the grayscale image to obtain a binary image; the specific process is:
[0053] Step 22: Filtering and preprocessing the binarized image to obtain a binarized image after removing clutter.
[0054] Other steps and parameters are the same as those in Embodiment 1.
specific Embodiment approach 3
[0055] Embodiment 3: The difference between this embodiment and Embodiment 1 or 2 is that in the step 21, the grayscale image is subjected to binarization preprocessing to obtain a binarization image; the specific process is:
[0056] The gray threshold segmentation algorithm is used to process each row of the gray image, and the gray threshold of each row is set between the maximum gray value of each row and the average gray value of the row, which can accurately segment the lane and the background, namely: RowAvg [i]<T[i]<MaxGray[i];
[0057] Among them: RowAvg[i] is the average gray value of row i; MaxGray[i] is the maximum gray value of row i; T[i] is the gray threshold of row i; the value of i is a positive integer, such as 240;
[0058] In order to simplify the complexity of the algorithm, select a scale factor R, 0<R<1, define
[0059] T[i]=RowAvg[i]+(MaxGray[i]-RowAvg[i])*R
[0060] * is the multiplication sign.
[0061] Other steps and parameters are the same as t...
PUM
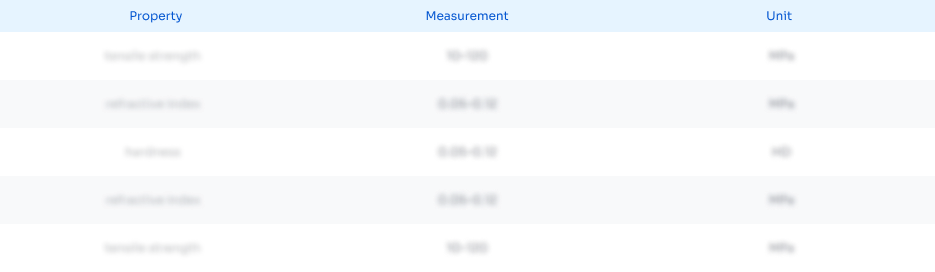
Abstract
Description
Claims
Application Information
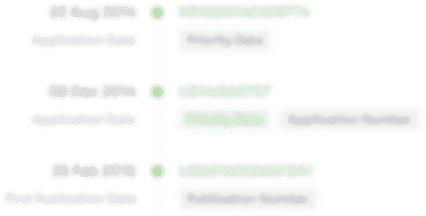
- R&D Engineer
- R&D Manager
- IP Professional
- Industry Leading Data Capabilities
- Powerful AI technology
- Patent DNA Extraction
Browse by: Latest US Patents, China's latest patents, Technical Efficacy Thesaurus, Application Domain, Technology Topic, Popular Technical Reports.
© 2024 PatSnap. All rights reserved.Legal|Privacy policy|Modern Slavery Act Transparency Statement|Sitemap|About US| Contact US: help@patsnap.com