ML-kNN (machine learning-k-nearest neighbor) improving method and ML-kNN improving system applicable to multi-label classification
A multi-label and label technology, which is applied in the field of ML-kNN improvement methods and systems, can solve the problems of not considering label correlation, decreasing label discrimination, increasing classification error, etc.
- Summary
- Abstract
- Description
- Claims
- Application Information
AI Technical Summary
Problems solved by technology
Method used
Image
Examples
Embodiment Construction
[0036] The invention provides an improved ML-kNN method suitable for multi-label classification, with the goal of improving the performance of ML-kNN in multi-label classification.
[0037] To achieve the above object, the technical scheme adopted in the present invention is as follows:
[0038] Step 1: Obtain the original data set, which includes multiple samples and involves a total of C label categories, where each sample has multi-class labels and multi-class features, and counts the total number of samples of each class of labels in the original data set , as the number of label samples, count the total number of samples of each type of feature in the samples of each type of label, as the number of feature samples, and calculate the feature label weight according to the number of label samples and the number of feature samples, where each feature corresponds to a feature value . Calculate the feature label weight, and its specific implementation method includes the follo...
PUM
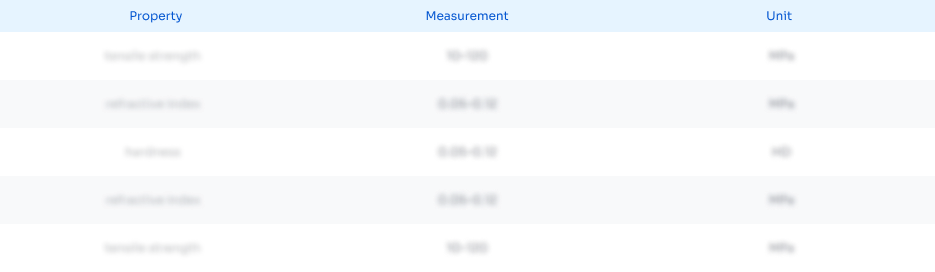
Abstract
Description
Claims
Application Information
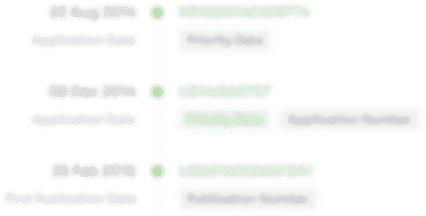
- R&D Engineer
- R&D Manager
- IP Professional
- Industry Leading Data Capabilities
- Powerful AI technology
- Patent DNA Extraction
Browse by: Latest US Patents, China's latest patents, Technical Efficacy Thesaurus, Application Domain, Technology Topic, Popular Technical Reports.
© 2024 PatSnap. All rights reserved.Legal|Privacy policy|Modern Slavery Act Transparency Statement|Sitemap|About US| Contact US: help@patsnap.com