Multi-layer aggregation for object detection
An object detection, object technology, applied in the field of machine learning, which can solve the problems of lack, no capture, bad local minimum back propagation optimization, etc.
- Summary
- Abstract
- Description
- Claims
- Application Information
AI Technical Summary
Problems solved by technology
Method used
Image
Examples
Embodiment Construction
[0017] The quality of one or more features is important for many image analysis tasks. Useful features can be constructed from raw data using machine learning. The involvement of machines can better distinguish or identify useful features than humans. Given the large number of possible features for images and the variety of image sources, machine learning methods are more robust than human programming. Machine learning to differentiate features can be applied to various products that rely on image analysis such as person re-identification or organ detection. Provides object detection (whether it is an organ, person re-identification, or other object) by leveraging the machine to learn discriminative features and train a classifier.
[0018] Provides a deep network framework for constructing features from raw image data. Rather than using only pre-programmed features (such as extracted Haar wavelets, hue-saturation value (HSV) histograms, or local binary patterns (LBP)), dee...
PUM
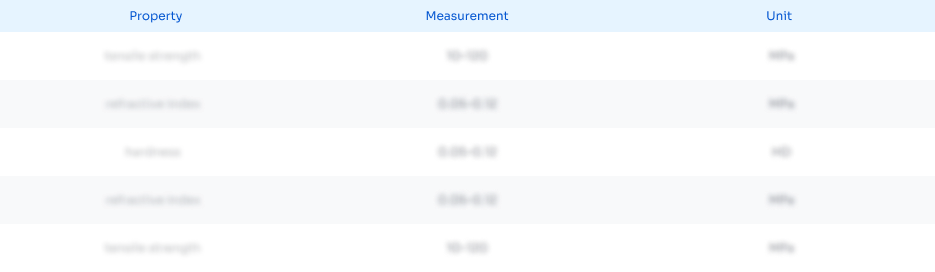
Abstract
Description
Claims
Application Information
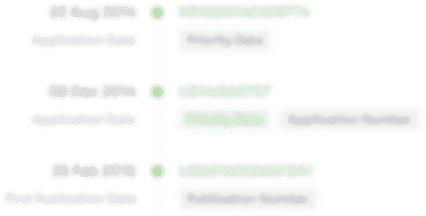
- R&D Engineer
- R&D Manager
- IP Professional
- Industry Leading Data Capabilities
- Powerful AI technology
- Patent DNA Extraction
Browse by: Latest US Patents, China's latest patents, Technical Efficacy Thesaurus, Application Domain, Technology Topic, Popular Technical Reports.
© 2024 PatSnap. All rights reserved.Legal|Privacy policy|Modern Slavery Act Transparency Statement|Sitemap|About US| Contact US: help@patsnap.com