Deep learning-based short-term traffic flow prediction method
A short-term traffic flow and deep learning technology, applied in the field of short-term traffic flow prediction, can solve the problems of not being able to make full use of the temporal and spatial characteristics and periodic characteristics of traffic flow data, and achieve the effect of reducing manpower consumption
- Summary
- Abstract
- Description
- Claims
- Application Information
AI Technical Summary
Problems solved by technology
Method used
Image
Examples
Embodiment Construction
[0032] The technical solution of the present invention will be specifically described below in conjunction with the accompanying drawings.
[0033] A short-term traffic flow prediction method based on deep learning of the present invention is specifically implemented according to the following steps,
[0034] Step S1: Consider the spatial correlation of traffic flow data, and obtain the spatial characteristics of traffic flow prediction points;
[0035] In this example, firstly, collect the traffic flow data set of the target detection point and its adjacent points, map the traffic flow data of the points in the area at the same time to a one-dimensional vector, take the predicted point as the reference point, and map the traffic flow data of the predicted point to The traffic flow data is placed in the center of the vector, and the north and south are used as the measurement standard. Set whether the data point in the vector is before or after the prediction point, with the n...
PUM
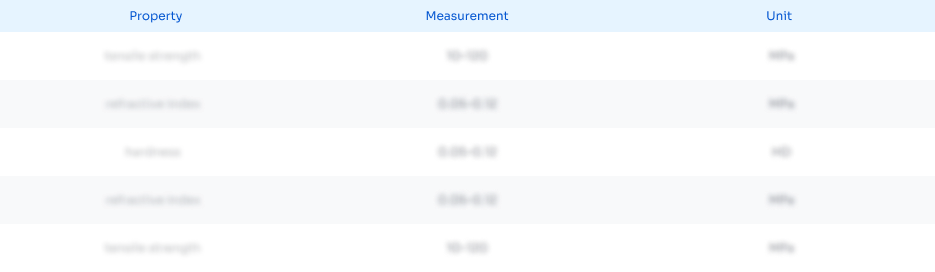
Abstract
Description
Claims
Application Information
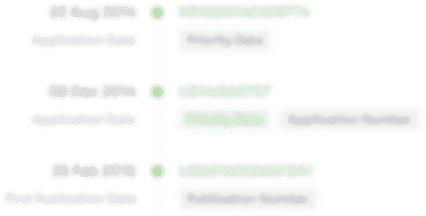
- R&D Engineer
- R&D Manager
- IP Professional
- Industry Leading Data Capabilities
- Powerful AI technology
- Patent DNA Extraction
Browse by: Latest US Patents, China's latest patents, Technical Efficacy Thesaurus, Application Domain, Technology Topic, Popular Technical Reports.
© 2024 PatSnap. All rights reserved.Legal|Privacy policy|Modern Slavery Act Transparency Statement|Sitemap|About US| Contact US: help@patsnap.com