Nuclear power device fault diagnosis method based on local linear embedding and K-nearest neighbor classifier
A nearest-neighbor classifier and local linear embedding technology, which is applied to instruments, computer components, character and pattern recognition, etc., can solve problems that cannot meet the requirements of nuclear power plant fault diagnosis, and achieve accurate results
- Summary
- Abstract
- Description
- Claims
- Application Information
AI Technical Summary
Problems solved by technology
Method used
Image
Examples
Embodiment Construction
[0039] The present invention will be further described below in conjunction with accompanying drawing example:
[0040] Software of the present invention is to be platform with Visual Studio 2010, adopts C# and Matlab to mix and write, wherein the dimensionality reduction feature extraction module of data is realized by Matlab, and its main function is:
[0041] After connecting the system, input the normal operation data of the nuclear power plant and typical fault data for training to obtain the manifold learning model and K-nearest neighbor classifier model, and then connect to the nuclear power plant for real-time fault diagnosis. Diagnosis results are displayed in the main interface of fault diagnosis in real time in the form of text and curves.
[0042] like figure 1 Shown, the fault diagnosis method based on local linear embedding and K-nearest neighbor classifier of the present invention, its steps are as follows:
[0043] (1) Obtain the operating data of the nuclear...
PUM
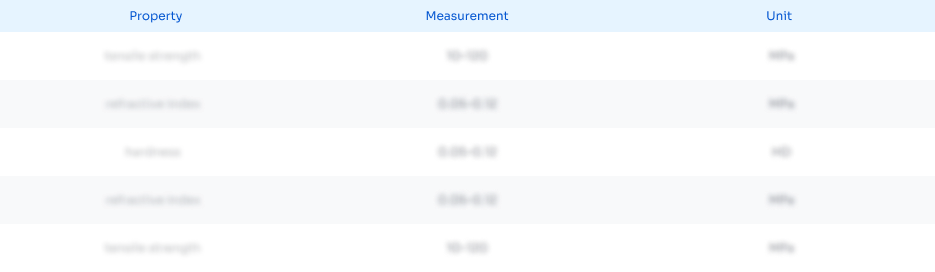
Abstract
Description
Claims
Application Information
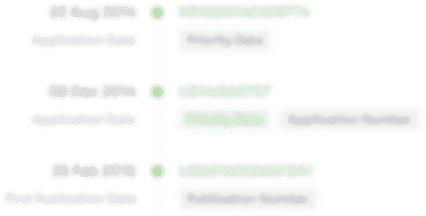
- R&D
- Intellectual Property
- Life Sciences
- Materials
- Tech Scout
- Unparalleled Data Quality
- Higher Quality Content
- 60% Fewer Hallucinations
Browse by: Latest US Patents, China's latest patents, Technical Efficacy Thesaurus, Application Domain, Technology Topic, Popular Technical Reports.
© 2025 PatSnap. All rights reserved.Legal|Privacy policy|Modern Slavery Act Transparency Statement|Sitemap|About US| Contact US: help@patsnap.com