Online Realization Method of Dialogue Policy Based on Multi-task Learning
A multi-task learning and strategy technology, applied in speech analysis, speech recognition, instruments, etc., can solve the problems of unstable learning rate, difficult to expand rules, and labor-intensive, etc., to achieve improved maintainability, stable training process, and strong construction The effect of modeling ability
- Summary
- Abstract
- Description
- Claims
- Application Information
AI Technical Summary
Problems solved by technology
Method used
Image
Examples
Embodiment Construction
[0032] Such as figure 1 As shown, the present embodiment follows the steps:
[0033] Step 101, acquire the corpus of the man-machine dialogue in real time from the online dialogue system.
[0034] In this embodiment, the process flow of the online spoken dialogue system for real-time acquisition of man-machine dialogue materials is as follows: figure 2 As shown, the steps of a complete dialogue flow include:
[0035] Step 201: Speech recognition, converting the user's voice into a text format;
[0036] Step 202: Semantic understanding, parsing the user voice text into semantics in the form of "slot-value pair";
[0037] Step 203: dialogue state tracking, updating current user state according to current information and historical information;
[0038] Step 204: the dialog strategy generates a reply action, taking the user's current state and user action as input, and generating a system reply action according to the dialog strategy;
[0039] Step 205: generating natural lan...
PUM
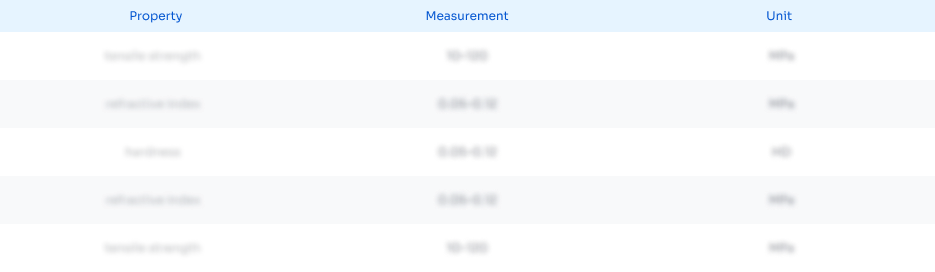
Abstract
Description
Claims
Application Information
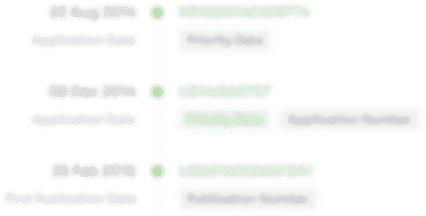
- R&D Engineer
- R&D Manager
- IP Professional
- Industry Leading Data Capabilities
- Powerful AI technology
- Patent DNA Extraction
Browse by: Latest US Patents, China's latest patents, Technical Efficacy Thesaurus, Application Domain, Technology Topic, Popular Technical Reports.
© 2024 PatSnap. All rights reserved.Legal|Privacy policy|Modern Slavery Act Transparency Statement|Sitemap|About US| Contact US: help@patsnap.com