Commodity purchase prediction modeling method
A modeling method and commodity technology, applied in the field of commodity purchase prediction modeling and machine learning modeling, can solve the problems of missing periodic rules and inability to grasp local changes in detail, to ensure robustness, scalability and practicability strong effect
- Summary
- Abstract
- Description
- Claims
- Application Information
AI Technical Summary
Problems solved by technology
Method used
Image
Examples
Embodiment
[0051] Example: 2015 Ali Mobile Recommendation
[0052] The selection methods in this embodiment include logistic regression (LR), random forest (RF), gradient boosting decision tree (GBDT), and neural network (NN). The evaluation data are all the results of single model tuning, and the characteristics, parameters, training prediction time and performance of each model are compared.
[0053] The feature selection of the model is mainly done by the GBDT and RF models. OOB samples (Out of Bag, samples not selected for training after random sampling) efficiently evaluate feature importance through permutation testing (using random arrangement of sample data for statistical inference). The feature importance also refers to the information gain of the feature, the calling frequency of the feature in different trees, etc. In special cases, operations such as feature knockout and value distribution inspection can be performed separately.
[0054] The comparison of algorithms in the...
PUM
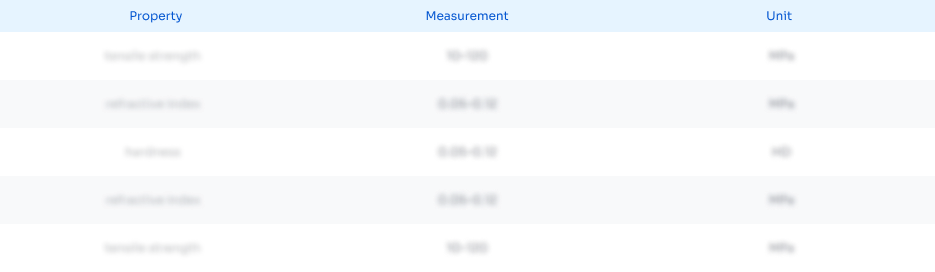
Abstract
Description
Claims
Application Information
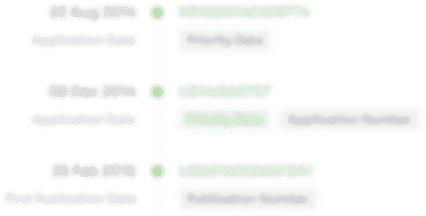
- R&D Engineer
- R&D Manager
- IP Professional
- Industry Leading Data Capabilities
- Powerful AI technology
- Patent DNA Extraction
Browse by: Latest US Patents, China's latest patents, Technical Efficacy Thesaurus, Application Domain, Technology Topic, Popular Technical Reports.
© 2024 PatSnap. All rights reserved.Legal|Privacy policy|Modern Slavery Act Transparency Statement|Sitemap|About US| Contact US: help@patsnap.com