Video compression method based on deep neural network
A deep neural network and video compression technology, applied in the field of video coding to achieve good scalability
- Summary
- Abstract
- Description
- Claims
- Application Information
AI Technical Summary
Problems solved by technology
Method used
Image
Examples
Embodiment Construction
[0027] In order to make the purpose, technical solution and advantages of the present invention clearer, the implementation method of the present invention will be further described in detail below in conjunction with the accompanying drawings.
[0028] A kind of video compression method based on deep neural network of the present embodiment, the steps are as follows:
[0029] (1) First collect and organize the required high-definition images (including Kodak lossless image library, ImageNet image library, etc.), organize standardized video image data sets, and construct neural network training data sets, test data sets and cross-validation sets.
[0030] (2) Establish a multi-layer prediction neural network and residual neural network: divide the image into non-overlapping M×N blocks, and train the prediction model of video coding mainly as intra-frame prediction mode and inter-frame prediction mode.
[0031] (3) For the inter-frame prediction mode, use the motion estimation ...
PUM
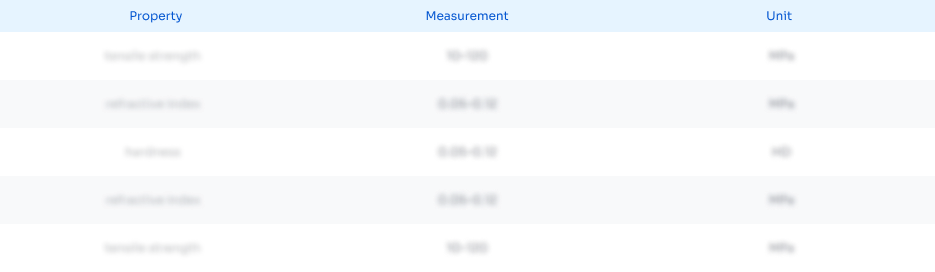
Abstract
Description
Claims
Application Information
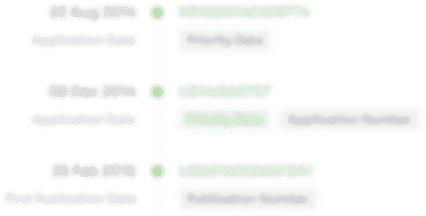
- R&D
- Intellectual Property
- Life Sciences
- Materials
- Tech Scout
- Unparalleled Data Quality
- Higher Quality Content
- 60% Fewer Hallucinations
Browse by: Latest US Patents, China's latest patents, Technical Efficacy Thesaurus, Application Domain, Technology Topic, Popular Technical Reports.
© 2025 PatSnap. All rights reserved.Legal|Privacy policy|Modern Slavery Act Transparency Statement|Sitemap|About US| Contact US: help@patsnap.com