Defect detection and classification method based on FCNs (fully convolutional networks) and applied to galvanized stamping parts
A convolutional neural network, defect detection technology, applied in the field of image processing and deep learning, can solve the problems of complex image processing process, difficult to meet engineering requirements, unstable image quality, etc., achieve high classification accuracy, good real-time performance, avoid Effects of preprocessing and feature extraction
- Summary
- Abstract
- Description
- Claims
- Application Information
AI Technical Summary
Problems solved by technology
Method used
Image
Examples
Embodiment Construction
[0029] Process flow of the present invention such as figure 1 As shown, first, collect samples containing various types of defects, calculate the standard deviation of the gray level of the samples, and distinguish the defective workpieces from the qualified workpieces according to the preliminary binary classification of the standard deviation; then, simple preprocessing of the initially screened samples improves Contrast, make the features more obvious, and extract the region of interest; use the processed samples as the input of the fully convolutional neural network for training; finally set the threshold for the output samples to judge the type of defects. The specific implementation process of the technical solution of the present invention will be described below in conjunction with the accompanying drawings.
[0030] 1. Collect defective samples;
[0031] Acquire sample images containing various types of blemishes.
[0032] 2. Preliminary two classifications based on...
PUM
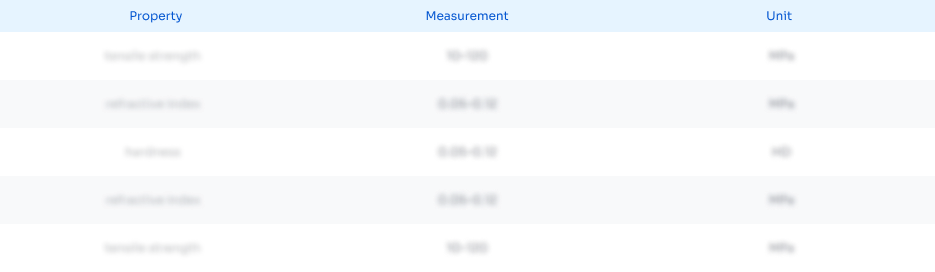
Abstract
Description
Claims
Application Information
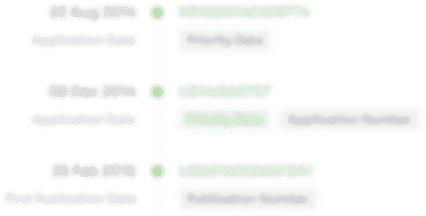
- R&D
- Intellectual Property
- Life Sciences
- Materials
- Tech Scout
- Unparalleled Data Quality
- Higher Quality Content
- 60% Fewer Hallucinations
Browse by: Latest US Patents, China's latest patents, Technical Efficacy Thesaurus, Application Domain, Technology Topic, Popular Technical Reports.
© 2025 PatSnap. All rights reserved.Legal|Privacy policy|Modern Slavery Act Transparency Statement|Sitemap|About US| Contact US: help@patsnap.com