A Method for Sentiment Polarity Analysis of Heterogeneous Migration Images Based on Multimodal Deep Latent Correlation
An emotional polarity and multi-modal technology, applied in the field of image content understanding and data analysis, can solve the problems that the effectiveness of image emotional analysis has not been fully proved, and the structural correlation between visual modality and text modality is ignored.
- Summary
- Abstract
- Description
- Claims
- Application Information
AI Technical Summary
Problems solved by technology
Method used
Image
Examples
Embodiment
[0104] refer to figure 1 , an emotional polarity analysis method for heterogeneous transfer images based on multimodal deep latent correlation, including the following steps:
[0105] 1) Construct an initial emotional image data set, use emotional vocabulary as keywords to obtain corresponding images from image sharing social networking sites, and then use the emotional polarity corresponding to the emotional vocabulary as the image emotional polarity label;
[0106] 2) Clear the noise data in the initial emotion image data set, and use the emotion consistency discrimination method and the probability sampling model based on the multimodal deep convolutional neural network to remove the noise;
[0107] 3) Construct a heterogeneous transfer model based on multimodal deep latent correlation, use this model to train source domain text and target domain image, and optimize until the mapping features of text and image in latent space are highly correlated;
[0108] 4) Construct a ...
PUM
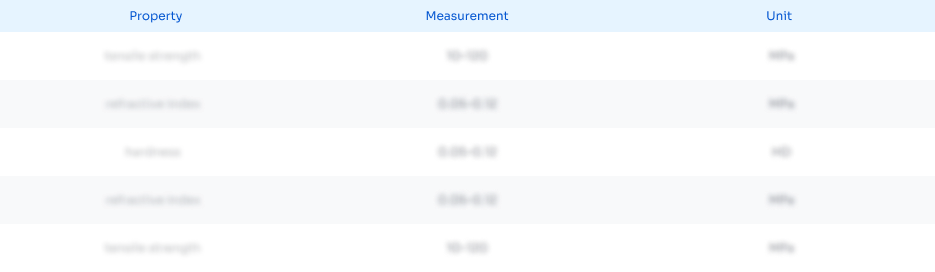
Abstract
Description
Claims
Application Information
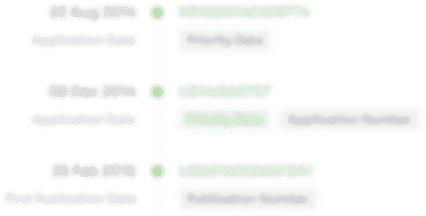
- R&D Engineer
- R&D Manager
- IP Professional
- Industry Leading Data Capabilities
- Powerful AI technology
- Patent DNA Extraction
Browse by: Latest US Patents, China's latest patents, Technical Efficacy Thesaurus, Application Domain, Technology Topic, Popular Technical Reports.
© 2024 PatSnap. All rights reserved.Legal|Privacy policy|Modern Slavery Act Transparency Statement|Sitemap|About US| Contact US: help@patsnap.com