Unmanned aerial vehicle landing landform image classification method based on DCT-CNN model
A technology of DCT-CNN and classification method, which is applied in the direction of biological neural network model, computer parts, character and pattern recognition, etc., can solve the problems of increased training model time, complex structure, and inconspicuous differences, so as to shorten the training time, Effects that are simple to calculate and less time-consuming
- Summary
- Abstract
- Description
- Claims
- Application Information
AI Technical Summary
Problems solved by technology
Method used
Image
Examples
Embodiment Construction
[0032] Embodiments of the present invention are described in detail below, examples of which are shown in the drawings, wherein the same or similar reference numerals designate the same or similar elements or elements having the same or similar functions throughout. The embodiments described below by referring to the figures are exemplary only for explaining the present invention and should not be construed as limiting the present invention.
[0033] like figure 1 Shown, according to the UAV landing landform image classification method based on DCT-CNN model of the present invention, comprise the following several steps:
[0034] Step S1: Obtain a training image set and a test image set of the UAV landing landform;
[0035] Step S2: DCT transformation of the UAV landform image, and screening of DCT coefficients;
[0036] After an image is transformed by DCT, most of the energy of the image is concentrated in the low-frequency DCT coefficients, so the low-frequency coefficien...
PUM
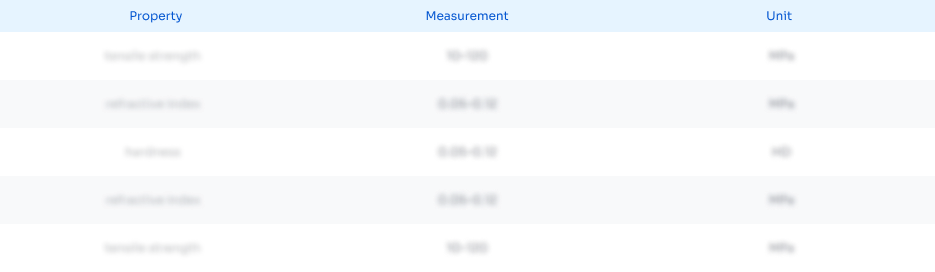
Abstract
Description
Claims
Application Information
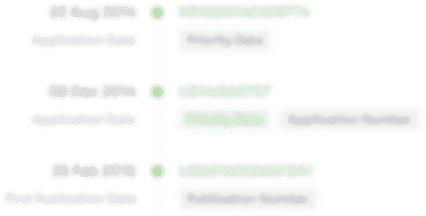
- R&D Engineer
- R&D Manager
- IP Professional
- Industry Leading Data Capabilities
- Powerful AI technology
- Patent DNA Extraction
Browse by: Latest US Patents, China's latest patents, Technical Efficacy Thesaurus, Application Domain, Technology Topic, Popular Technical Reports.
© 2024 PatSnap. All rights reserved.Legal|Privacy policy|Modern Slavery Act Transparency Statement|Sitemap|About US| Contact US: help@patsnap.com