Convolutional neural network feature extraction method based on principal component analysis
A convolutional neural network and principal component analysis technology, applied in neural learning methods, biological neural network models, neural architectures, etc., to achieve rich semantic information, easy use, and noise elimination.
- Summary
- Abstract
- Description
- Claims
- Application Information
AI Technical Summary
Problems solved by technology
Method used
Image
Examples
Embodiment Construction
[0018] combine figure 1 , the present invention is based on the feature extraction method of convolutional neural network based on principal component analysis, which uses principal component analysis to process the features of the middle layer of convolutional neural network to obtain the feature after dimensionality reduction, that is, through the convolutional neural network, the efficient and refined The depth features of , the steps are as follows:
[0019] 1. Select the type of convolutional neural network and perform image classification training on the Imagenet dataset. You can also select a trained convolutional neural network model and remove the fully connected layer and softmax layer of the convolutional neural network.
[0020] 2. Select the image to extract features, according to the selected convolutional neural network, normalize the image according to the requirements of the corresponding convolutional neural network, and adjust its size to the input size requ...
PUM
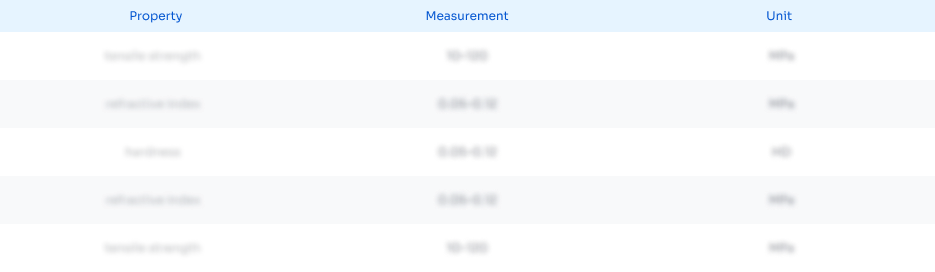
Abstract
Description
Claims
Application Information
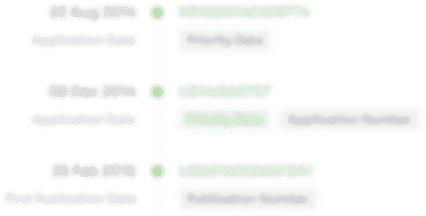
- R&D Engineer
- R&D Manager
- IP Professional
- Industry Leading Data Capabilities
- Powerful AI technology
- Patent DNA Extraction
Browse by: Latest US Patents, China's latest patents, Technical Efficacy Thesaurus, Application Domain, Technology Topic, Popular Technical Reports.
© 2024 PatSnap. All rights reserved.Legal|Privacy policy|Modern Slavery Act Transparency Statement|Sitemap|About US| Contact US: help@patsnap.com