Image classification method based on locality constraints and saliency
A classification method and local technology, applied in the field of computer vision, to achieve the effect of improved classification results and strong discriminative power
- Summary
- Abstract
- Description
- Claims
- Application Information
AI Technical Summary
Problems solved by technology
Method used
Image
Examples
Embodiment 1
[0050] In this embodiment, the image classification method based on locality constraints and saliency includes two parts: a training process 100 and a testing process 120 .
[0051] In the training process 100, step 101 is executed to select a certain number of images to construct a training set D, and the corresponding class label set is L. Step 102 is executed to extract the local feature descriptors of each image in the training set to form a set Y of local feature descriptors. Step 103 is executed to cluster the set Y of local feature descriptors in the training set to obtain a visual word dictionary B, where M is the number of visual words. Step 104 is executed to extract local feature descriptors for each training image, and the set is X. Execute step 105, perform locality-constrained encoding calculations on each training image, and the encoding formula based on locality constraints is: where x i Indicates the i-th local feature descriptor; b j Indicates the jth vi...
Embodiment 2
[0055] When encoding an image, it is intuitively understood that the significant part of the encoded value should be given a greater weight to highlight the role of this part of the feature in the image representation. The description of the idea is as follows: figure 2 Shown is the original image, Figure 2a Shown is the saliency map corresponding to the original image. exist Figure 2b The black dots in represent the local feature descriptors in the background to be encoded, such as Figure 2c As shown, the diagram shows the coding situation of black dots in background pixels before the introduction of salience, such as Figure 2d As shown, the figure shows the encoding of background pixel black dots after the introduction of salience. It can be seen that for the background pixel black dots, due to the low saliency value, the encoding value after the introduction of salience is higher than that before the introduction of salience. Coded values have been reduced. exis...
Embodiment 3
[0057]The experimental image databases include Oxford 17 flower database, Oxford 102 flower database, Caltech 101, Caltech 256 and UIUC8 database. The local features used in the experiment are 128-dimensional SIFT feature descriptors, the sampling interval is 8 pixels, and the area blocks around the descriptors are 16×16 pixels. Each image library obtains a dictionary containing 400 visual words through the k_means method; 30 images are randomly selected for each type of image as training, and the remaining images are used as testing. In the experiment, the DRFI salient region extraction method is used to obtain the saliency map of the image. In the experiment, the parameter β is equal to 10, and the number of nearest neighbors is 5. The experimental results are shown in Table 1. In the table, the LLC method is used to encode and calculate the Oxford 17 flower library, Oxford 102 flower library, Caltech 101, Caltech 256 and UIUC8 library, and the obtained visual word coding ...
PUM
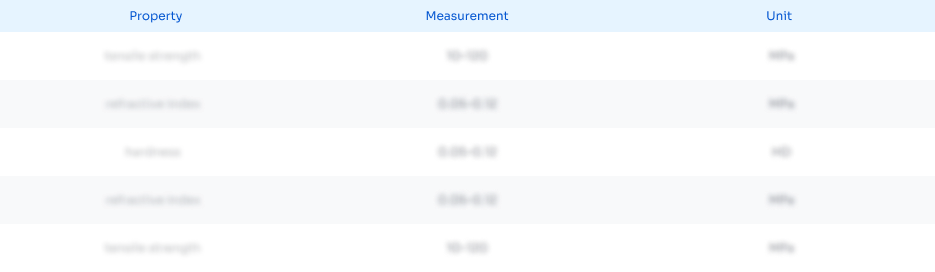
Abstract
Description
Claims
Application Information
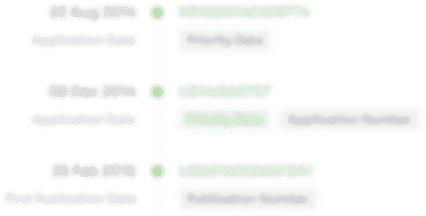
- R&D
- Intellectual Property
- Life Sciences
- Materials
- Tech Scout
- Unparalleled Data Quality
- Higher Quality Content
- 60% Fewer Hallucinations
Browse by: Latest US Patents, China's latest patents, Technical Efficacy Thesaurus, Application Domain, Technology Topic, Popular Technical Reports.
© 2025 PatSnap. All rights reserved.Legal|Privacy policy|Modern Slavery Act Transparency Statement|Sitemap|About US| Contact US: help@patsnap.com