A multi-view classifier and design method based on local features
A local feature and multi-view technology, applied in the field of pattern recognition, can solve the problems of effective data information enhancement, low performance of related classifiers, performance limitations of effective data information classifiers, etc., and achieve the effect of improving classification performance
- Summary
- Abstract
- Description
- Claims
- Application Information
AI Technical Summary
Problems solved by technology
Method used
Image
Examples
Embodiment Construction
[0038] The present invention will be further elaborated below by describing a preferred specific embodiment in detail in conjunction with the accompanying drawings.
[0039] Such as figure 1 As shown, the present invention discloses a multi-view classifier based on local features, which is a model implemented by Matlab language, which includes an unlabeled multi-view large data set generation module 1, global and local structural risk minimization classification implement module 2 and multi-view data local feature extraction module 3, in the present embodiment, also comprise a multi-view data collection module, can be from UCI machine learning library (http: / / archive.ics.uci.edu / ml / ) collects multi-view data and transmits the data to an unlabeled multi-view large data set generation module 1, a global and local structural risk minimization classifier implementation module 2, and a multi-view data local feature extraction module 3. The collection module essentially collects l...
PUM
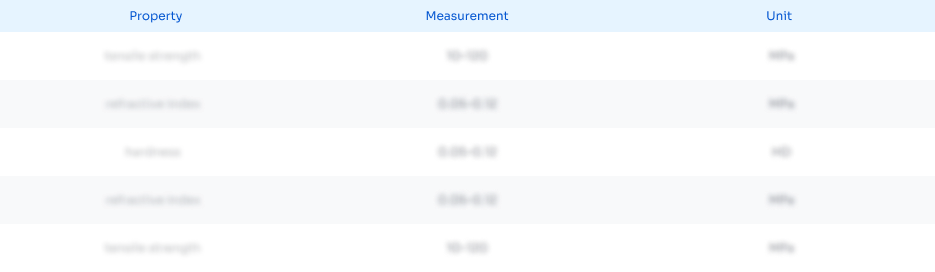
Abstract
Description
Claims
Application Information
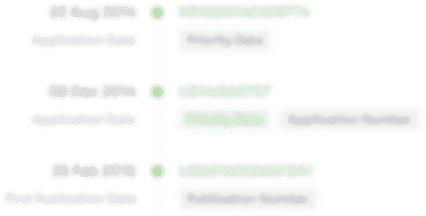
- R&D
- Intellectual Property
- Life Sciences
- Materials
- Tech Scout
- Unparalleled Data Quality
- Higher Quality Content
- 60% Fewer Hallucinations
Browse by: Latest US Patents, China's latest patents, Technical Efficacy Thesaurus, Application Domain, Technology Topic, Popular Technical Reports.
© 2025 PatSnap. All rights reserved.Legal|Privacy policy|Modern Slavery Act Transparency Statement|Sitemap|About US| Contact US: help@patsnap.com