Image classification method based on low-rank sparse representation
A technology of sparse representation and classification method, applied in the field of image recognition, it can solve the problem of not being able to process new samples, and achieve the effect of strong robustness
- Summary
- Abstract
- Description
- Claims
- Application Information
AI Technical Summary
Problems solved by technology
Method used
Image
Examples
Embodiment Construction
[0043]An image classification method based on low-rank sparse representation, including the following steps:
[0044] Step 1, from the C class training sample matrix A=[A 1 ,A 2 ,...,A c ]∈R m×N , test sample Y=[y 1 ,y 2 ,...,y M ]∈R m×M , normalize each column of the training sample A and the test sample Y to the unit L 2 Norm, where N represents the number of training samples, M represents the number of test samples, and m represents the dimension of the sample.
[0045] Step 2. Calculate the projection matrix P:
[0046] Step 2.1, initialization: make parameters λ>0, γ>0, α>0, η>0, Z 0 =W 0 =E 0 =Y 10 =Y 20 =0,P 0 is a random matrix, μ 0 =0.1,μ max =10 10 , ρ=1.1, ε=10 -3 , maxiter=1000, k=0, where μ is the penalty parameter, k is the number of iterations, η and α are balance factors, Z, W and E are coefficient matrices, Y 1k , Y 2k is the Lagrangian multiplier;
[0047] Step 2.2, use the formula (1) to update the coefficient matrix Z:
[0048]
[0...
PUM
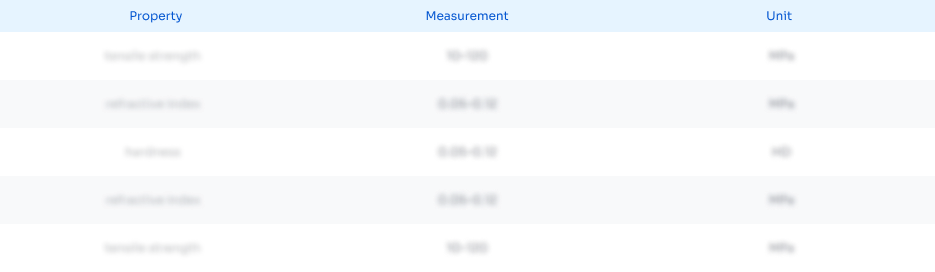
Abstract
Description
Claims
Application Information
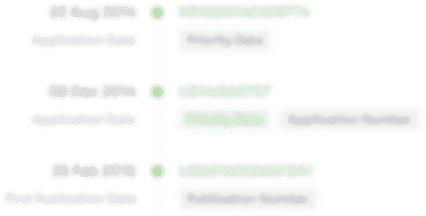
- R&D Engineer
- R&D Manager
- IP Professional
- Industry Leading Data Capabilities
- Powerful AI technology
- Patent DNA Extraction
Browse by: Latest US Patents, China's latest patents, Technical Efficacy Thesaurus, Application Domain, Technology Topic, Popular Technical Reports.
© 2024 PatSnap. All rights reserved.Legal|Privacy policy|Modern Slavery Act Transparency Statement|Sitemap|About US| Contact US: help@patsnap.com