Modified local sensitive hash vehicle retrieval method based on multitask deep learning
A local sensitive hash and deep learning technology, applied in neural learning methods, computer components, special data processing applications, etc., can solve problems such as low level of automation and intelligence, speeding up retrieval speed, and difficulty in meeting image retrieval needs.
- Summary
- Abstract
- Description
- Claims
- Application Information
AI Technical Summary
Problems solved by technology
Method used
Image
Examples
Embodiment Construction
[0113] The following will clearly and completely describe the technical solutions in the embodiments of the present invention with reference to the drawings in the embodiments of the present invention.
[0114] refer to Figure 1 to Figure 5 , a modified local sensitive hash vehicle retrieval method based on multi-task deep learning, the overall flow chart is as follows figure 1 As shown, first, the pictures in the database are sent to the multi-task end-to-end convolutional neural network for deep learning and training recognition, and a large number of training and layer-by-layer progressive network structures are used to deeply learn various attribute information of vehicles, including Model, car series, car logo, color, license plate; then use this convolutional network to extract the vehicle attribute hash code learned in parallel by segmenting the vehicle image, and extract the instance features of the vehicle from the constructed feature pyramid module; retrieve the veh...
PUM
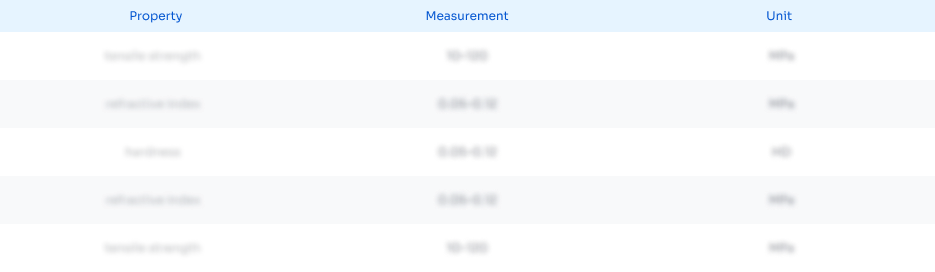
Abstract
Description
Claims
Application Information
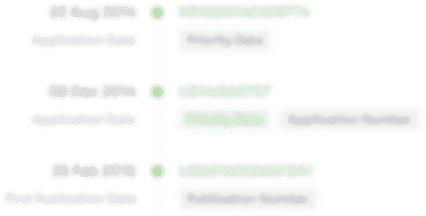
- R&D Engineer
- R&D Manager
- IP Professional
- Industry Leading Data Capabilities
- Powerful AI technology
- Patent DNA Extraction
Browse by: Latest US Patents, China's latest patents, Technical Efficacy Thesaurus, Application Domain, Technology Topic, Popular Technical Reports.
© 2024 PatSnap. All rights reserved.Legal|Privacy policy|Modern Slavery Act Transparency Statement|Sitemap|About US| Contact US: help@patsnap.com