Spatial-spectral weighted TV-based hyperspectral-image restoration method of non-convex low-rank relaxation
A hyperspectral image and restoration method technology, applied in the field of image restoration, can solve the problems of poor Gaussian noise effect, lack of spatial constraints, neglect of spectral similarity, etc., achieve high unbiasedness and robustness, protect texture information, and enhance The effect of the slice smoothness property
- Summary
- Abstract
- Description
- Claims
- Application Information
AI Technical Summary
Problems solved by technology
Method used
Image
Examples
Embodiment Construction
[0014] The concrete steps that realize content of the present invention are:
[0015] Step 1: Input the noise-contaminated hyperspectral image Y ∈ R M×N×P , decompose the hyperspectral image Y to get Y=X+S+N, where X∈R M×N×P For the original clean hyperspectral image, S ∈ R M×N×P It is sparse noise, including strip noise, impulse noise, etc., N∈R M×N×P is a Gaussian noise image, M and N are the spatial dimensions, and P is the spectral dimension;
[0016] Step 2: First use the weighted l 1 Norm strategy, for hyperspectral TV (HTV, Hyperspectral TV), reweight to construct weighted TV (Weighted HTV, WHTV):
[0017]
[0018] where G∈R M×N is the synthetic gradient, j represents the number of bands, i represents a spatial point, and g i is the i-th element in G, W∈R M×N is the spatial weight matrix, w i is the i-th pixel of the spatial weight W, ⊙ is the Hardamard product, representing the component product, w i Commonly used forms are:
[0019]
[0020] mu 1 is...
PUM
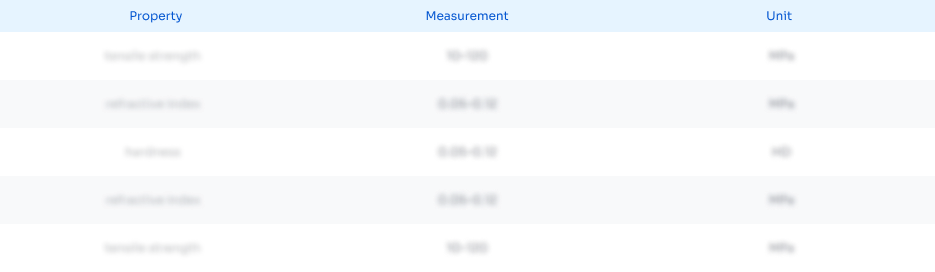
Abstract
Description
Claims
Application Information
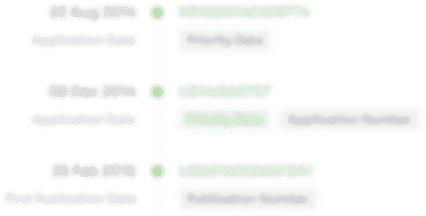
- R&D Engineer
- R&D Manager
- IP Professional
- Industry Leading Data Capabilities
- Powerful AI technology
- Patent DNA Extraction
Browse by: Latest US Patents, China's latest patents, Technical Efficacy Thesaurus, Application Domain, Technology Topic, Popular Technical Reports.
© 2024 PatSnap. All rights reserved.Legal|Privacy policy|Modern Slavery Act Transparency Statement|Sitemap|About US| Contact US: help@patsnap.com