While drilling measurement signal processing method based on wavelet denoising and neural network identification
A technology of neural network identification and measurement while drilling, applied in neural learning method, biological neural network model, measurement and other directions, can solve the problem of inability to realize measurement while drilling signal processing, etc., and achieve the effect of strong signal recognition ability and high processing speed.
- Summary
- Abstract
- Description
- Claims
- Application Information
AI Technical Summary
Problems solved by technology
Method used
Image
Examples
Embodiment 1
[0046] Such as figure 1 shown.
[0047] A measurement-while-drilling signal processing method based on wavelet denoising and neural network identification, which processes the noisy signal obtained by simulating downhole parameters, including the following steps:
[0048]1) Analyze the downhole signal containing noise; transform the time domain signal into a frequency domain signal by fast Fourier transform to obtain the frequency distribution of the signal, and then obtain the frequency distribution of the useful signal and the frequency distribution of the noise signal to prepare for the filtering operation ;Such as figure 2 , Figure 4 shown; by observation Figure 4 It can be seen that there are signals around the frequency 0~0.8Hz (useful signal), 1.2Hz, 2.3Hz, 3.3Hz, 4.4Hz and 6.5Hz;
[0049] 2) Denoising the downhole signal containing noise by wavelet transform to obtain the denoising signal; the specific method of denoising the downhole signal containing noise by ...
Embodiment 2
[0054] The measurement-while-drilling signal processing method based on wavelet denoising and neural network identification as described in Embodiment 1, further, the BP neural network in the step 3) is a multi-layer feed-forward neural network, and the multi-layer feed-forward The neural network includes an input layer, a hidden layer and an output layer; the transfer function of the BP neural network is a logarithmic S-type function, that is, y=logsig(x); y∈(0,1); where, x is any input data, y is the corresponding output data, and the value range of y is 0~1.
Embodiment 3
[0056] Such as Figure 7 shown.
[0057] The measurement-while-drilling signal processing method based on wavelet denoising and neural network identification as described in Embodiment 2, further,
[0058] 3.1) The forward propagation process of the signal:
[0059] 3.1.1) The input of the i-th node in the hidden layer Among them, X1 is the input of the input layer, ω i,j is the weight of the input layer, i represents the i-th node of the hidden layer, and j represents the j-th node of the input layer; β n Is the threshold of the hidden layer, Y1 is the output of the output layer; M=9; n=1,2,3...M;
[0060] 3.1.2) The output of the i-th node in the hidden layer is O i = θ(net(i));
[0061] 3.1.3) The output Y1 of the output layer=φ[∑(O i · ω k,i )+a1]; k represents the kth node of the output layer; the value range of Y1 is (0,1), and a1 is the threshold of the output layer; the independent variable ω in the summation symbol k,i is the threshold between the hidden lay...
PUM
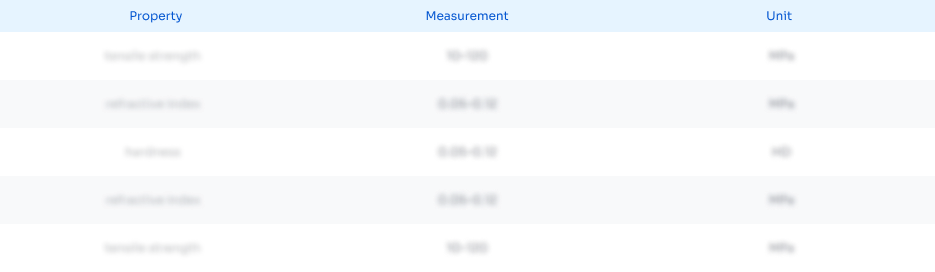
Abstract
Description
Claims
Application Information
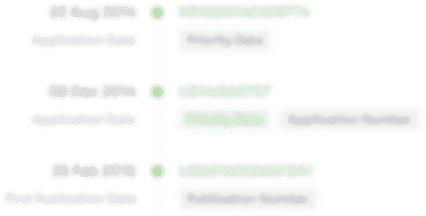
- R&D Engineer
- R&D Manager
- IP Professional
- Industry Leading Data Capabilities
- Powerful AI technology
- Patent DNA Extraction
Browse by: Latest US Patents, China's latest patents, Technical Efficacy Thesaurus, Application Domain, Technology Topic, Popular Technical Reports.
© 2024 PatSnap. All rights reserved.Legal|Privacy policy|Modern Slavery Act Transparency Statement|Sitemap|About US| Contact US: help@patsnap.com