Transaction index abnormality monitoring method based on deep learning model LSTM
A technology of deep learning and indicators, which is applied in the fields of instruments, finance, and data processing applications. It can solve problems such as inaccurate capture of abnormalities, incomplete coverage of abnormal index values, and prone to errors in the judgment of irregular time series indicators, etc., to achieve pre-judgment The effect of improving accuracy, improving efficiency, and saving the workload of manual analysis
- Summary
- Abstract
- Description
- Claims
- Application Information
AI Technical Summary
Problems solved by technology
Method used
Image
Examples
Embodiment Construction
[0034] In order to make the object, technical solution and advantages of the present invention clearer, the present invention will be further described in detail below in conjunction with the accompanying drawings and embodiments. It should be understood that the specific embodiments described here are only used to explain the present invention, not to limit the present invention.
[0035] A method for monitoring abnormal transaction indicators based on deep learning model LSTM, the method steps are:
[0036] Step 1. Collect and process the historical data of trading indicators, specifically:
[0037] Store the data of a certain index in the text or database according to the same time interval, such as: 1 minute or 5 minutes, and each data storage record is: value, time;
[0038] Collect and store the historical data of transaction indicators, clean them, and remove abnormal values, such as NA, null, or values that do not conform to business logic, such as: negative values;...
PUM
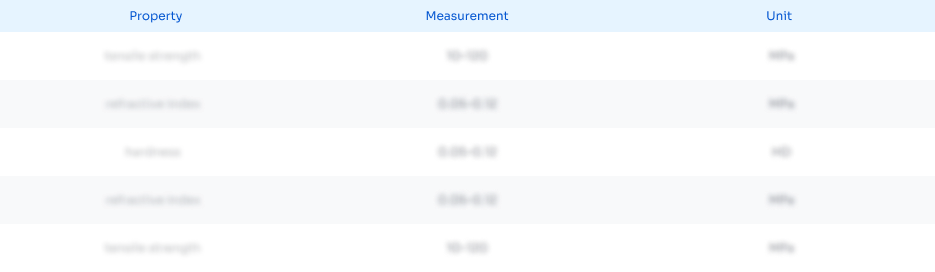
Abstract
Description
Claims
Application Information
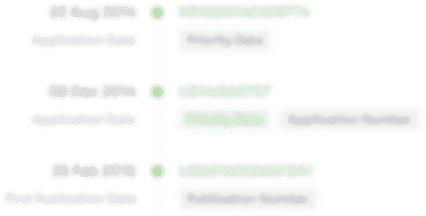
- R&D Engineer
- R&D Manager
- IP Professional
- Industry Leading Data Capabilities
- Powerful AI technology
- Patent DNA Extraction
Browse by: Latest US Patents, China's latest patents, Technical Efficacy Thesaurus, Application Domain, Technology Topic, Popular Technical Reports.
© 2024 PatSnap. All rights reserved.Legal|Privacy policy|Modern Slavery Act Transparency Statement|Sitemap|About US| Contact US: help@patsnap.com