Bearing fault mode diagnosis method and system based on deep learning
A failure mode, deep learning technology, applied in neural learning methods, mechanical bearing testing, mechanical component testing, etc., can solve problems such as affecting diagnostic results, non-stationary vibration signals that do not conform to FFT stationarity assumptions, etc., and achieve important practical performance Effect
- Summary
- Abstract
- Description
- Claims
- Application Information
AI Technical Summary
Problems solved by technology
Method used
Image
Examples
Embodiment Construction
[0031] Embodiments of the present invention are described in detail below, examples of which are shown in the drawings, wherein the same or similar reference numerals designate the same or similar elements or elements having the same or similar functions throughout. The embodiments described below by referring to the figures are exemplary and are intended to explain the present invention and should not be construed as limiting the present invention.
[0032] The method and system for diagnosing bearing fault modes based on deep learning according to embodiments of the present invention will be described below with reference to the accompanying drawings. First, the method for diagnosing bearing fault modes based on deep learning according to embodiments of the present invention will be described with reference to the accompanying drawings.
[0033] figure 1It is a flowchart of a method for diagnosing bearing fault modes based on deep learning in an embodiment of the present inv...
PUM
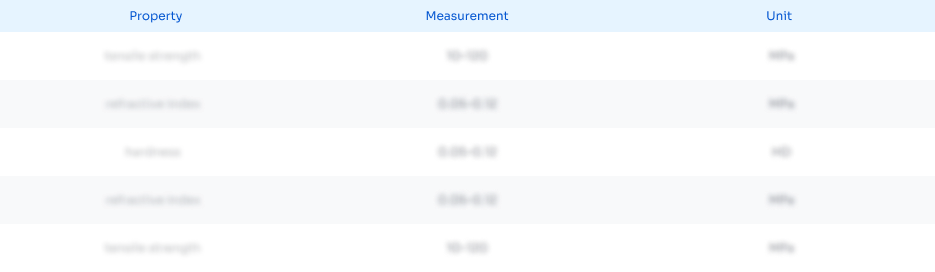
Abstract
Description
Claims
Application Information
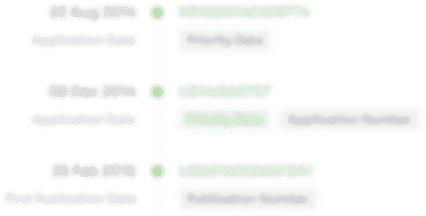
- R&D Engineer
- R&D Manager
- IP Professional
- Industry Leading Data Capabilities
- Powerful AI technology
- Patent DNA Extraction
Browse by: Latest US Patents, China's latest patents, Technical Efficacy Thesaurus, Application Domain, Technology Topic.
© 2024 PatSnap. All rights reserved.Legal|Privacy policy|Modern Slavery Act Transparency Statement|Sitemap