Zero-sample classifying method based on class transfer
A classification method and category technology, applied in computer parts, character and pattern recognition, instruments, etc., can solve the problems of heavy manual labeling workload, difficult to obtain training samples, and low efficiency.
- Summary
- Abstract
- Description
- Claims
- Application Information
AI Technical Summary
Problems solved by technology
Method used
Image
Examples
Embodiment Construction
[0031] A zero-shot classification method based on category transfer of the present invention will be described in detail below with reference to the embodiments and the accompanying drawings.
[0032] A zero-shot classification method based on category transfer of the present invention starts from the perspective of classifier learning, and uses sample semantic relations to realize knowledge transfer between different category classifiers, so that the known category classifiers learned in the training stage can be used Make reasonable label predictions for samples of unknown classes.
[0033] The invention is suitable for solving the problem of cross-modal zero-sample learning. The present invention represents features from two different modalities with visual features and semantic features, with X=[x 1 ,...,x i ,...,x N ]∈R p×N Represents the visual feature space of N samples from C known categories in the training phase, where p represents the dimension of visual feature...
PUM
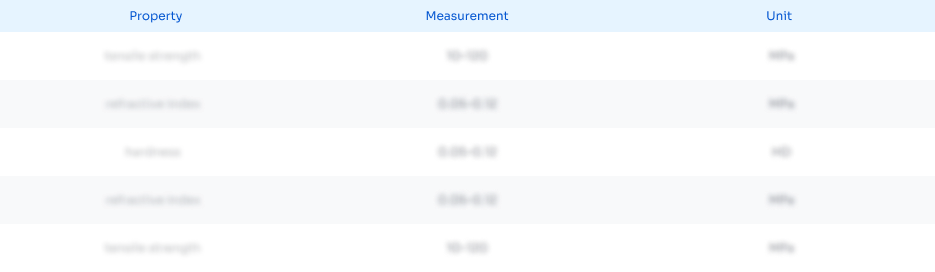
Abstract
Description
Claims
Application Information
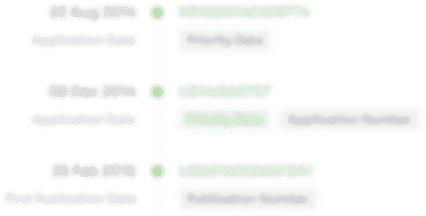
- Generate Ideas
- Intellectual Property
- Life Sciences
- Materials
- Tech Scout
- Unparalleled Data Quality
- Higher Quality Content
- 60% Fewer Hallucinations
Browse by: Latest US Patents, China's latest patents, Technical Efficacy Thesaurus, Application Domain, Technology Topic, Popular Technical Reports.
© 2025 PatSnap. All rights reserved.Legal|Privacy policy|Modern Slavery Act Transparency Statement|Sitemap|About US| Contact US: help@patsnap.com