Semi-supervised biomedical text semantic disambiguation method
A biomedical and semi-supervised technology, applied in the field of natural language processing semantic disambiguation, can solve problems such as weak globality, high cost, and difficulty in manual annotation, and achieve the effect of solving weak globality and improving accuracy.
- Summary
- Abstract
- Description
- Claims
- Application Information
AI Technical Summary
Problems solved by technology
Method used
Image
Examples
Embodiment Construction
[0039] (1) The user inputs biomedical text and generates sentence vector features
[0040] First, divide the biomedical text into phrases, then use the Word2Vec model to generate word vectors for the phrases, and then input the word vectors in each sentence into the two-way long-term and short-term memory model, and the model will output two sentence vectors, respectively with , form a new sentence vector by cascading :
[0041]
[0042] The new sentence vector is then input into the multi-layer perceptron get the final sentence vector :
[0043]
[0044]
[0045] .
[0046] (2) Use the label transfer method to automatically label unlabeled data and disambiguate ambiguous words
[0047] Use the sentence vector feature obtained in (1) as a vector graph node, calculate the similarity of each node, automatically propagate the most similar label for unlabeled data according to the label transfer method, and for ambiguous words, also transfer the most suitable...
PUM
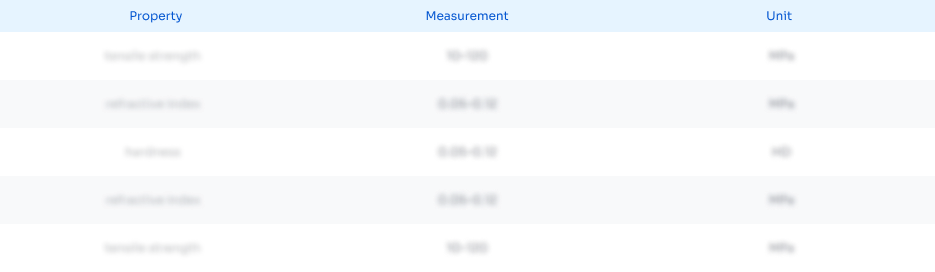
Abstract
Description
Claims
Application Information
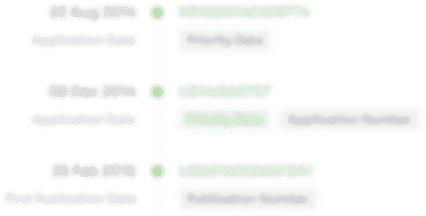
- R&D Engineer
- R&D Manager
- IP Professional
- Industry Leading Data Capabilities
- Powerful AI technology
- Patent DNA Extraction
Browse by: Latest US Patents, China's latest patents, Technical Efficacy Thesaurus, Application Domain, Technology Topic, Popular Technical Reports.
© 2024 PatSnap. All rights reserved.Legal|Privacy policy|Modern Slavery Act Transparency Statement|Sitemap|About US| Contact US: help@patsnap.com