Multi-work-condition rotary machine fault diagnosis method
A technology for rotating machinery and fault diagnosis, which is applied in the testing of machines/structural components, instruments, and data processing applications. It can solve problems such as frequent faults, difficult manual diagnosis, strong nonlinearity, etc., and achieve enhanced extraction capabilities and improved classification. Accuracy, the effect of improving linear separability
- Summary
- Abstract
- Description
- Claims
- Application Information
AI Technical Summary
Problems solved by technology
Method used
Image
Examples
Embodiment 1
[0032] Such as figure 1 As shown, a multi-condition rotating machinery fault diagnosis method, including:
[0033] S1. Collect and obtain the original time-domain vibration data matrix of the rotating machinery;
[0034] S2. Perform Fourier transform and normalization processing on the original time-domain vibration data matrix in turn to obtain a normalized amplitude-frequency vibration data matrix;
[0035] S3. Input the normalized amplitude-frequency vibration data matrix into the fault diagnosis model established by the serial combination of the convolutional neural network and the K-nearest neighbor classifier, and obtain the diagnosis result.
[0036] The process of step S1 specifically includes collecting the original time-domain vibration signals at the vibration monitoring points of the rotating machinery. The vibration monitoring points include rolling bearings, motor rotors, couplings, gears, etc. related structures, such as bearing supports, gearbox covers, etc. ...
Embodiment 2
[0076] This embodiment will further refine the description of the technical solution in combination with specific data, as follows:
[0077] S1. Collect the original vibration data matrix of the rotating machinery. In this embodiment, collect the original time-domain vibration signals at the vibration monitoring points of the rotating machinery, and arrange the time-domain vibration data into a time-domain vibration data matrix according to the sampling channels:
[0078]
[0079] In this embodiment, the single-channel radial vibration of the rolling bearing is used as the vibration data. Among them, a set of time domain vibration data contains 3 power frequency cycles, the sampling frequency is 12kHz, and the sampling time is 0.1s. According to the number of channels, the dimension of the time-domain vibration data matrix formed by it is 1×1200.
[0080] S2. Perform Fourier transform and normalization processing on the original vibration data in sequence to obtain a norma...
PUM
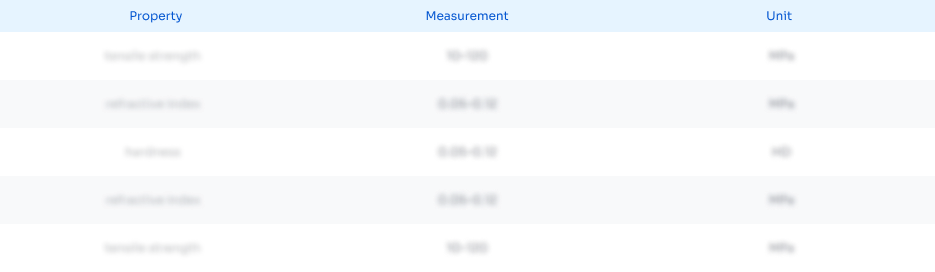
Abstract
Description
Claims
Application Information
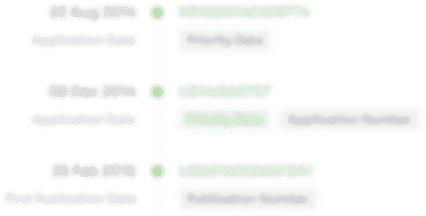
- R&D Engineer
- R&D Manager
- IP Professional
- Industry Leading Data Capabilities
- Powerful AI technology
- Patent DNA Extraction
Browse by: Latest US Patents, China's latest patents, Technical Efficacy Thesaurus, Application Domain, Technology Topic.
© 2024 PatSnap. All rights reserved.Legal|Privacy policy|Modern Slavery Act Transparency Statement|Sitemap