A Clustering Method for Industrial Process Data Based on Density Peak Clustering
An industrial process and data clustering technology, applied in the fields of instrument, calculation, character and pattern recognition, etc., can solve the problem that the number of cluster centers cannot be automatically determined, the optimal cluster center cannot be determined, and the accuracy of clustering results is low. problem, to achieve the effect of good applicability, reduced calculation amount, and high classification accuracy
- Summary
- Abstract
- Description
- Claims
- Application Information
AI Technical Summary
Problems solved by technology
Method used
Image
Examples
Embodiment 1
[0052] Embodiment 1 adopts the industrial process data clustering method based on the improved Density Peaks Clustering (DPC: Density Peaks Clustering) provided by the present invention, and verifies through the industrial process of semiconductors. Table 1 shows 16 different modalities and Correspondence table for industrial process data.
[0053] Table 1
[0054] modal Data points corresponding to the modality 1 1-24 2 25-49 3 50-73 4 74-82 5 83-107 6 108-132 7 133-153 8 154-178 9 179-203 10 204-223 11 224-248 12 249-269 13 270-294 14 295-318 15 319-340 16 341-364
[0055] The verification data used in Embodiment 1 of the present invention comes from the data of the semiconductor industry process, and the modern semiconductor production line is composed of hundreds of continuous batch processing stages. Each stage includes many steps carried out by expensive tools monitored by nu...
PUM
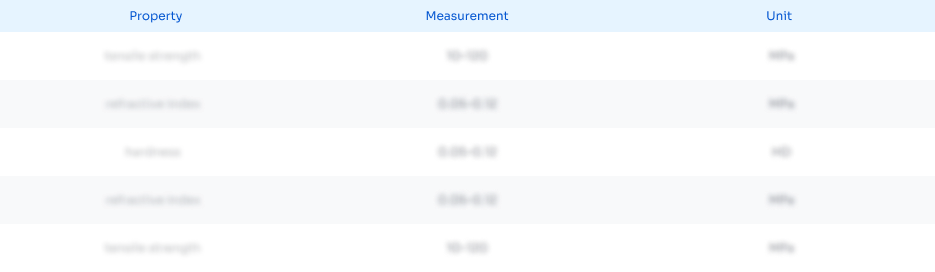
Abstract
Description
Claims
Application Information
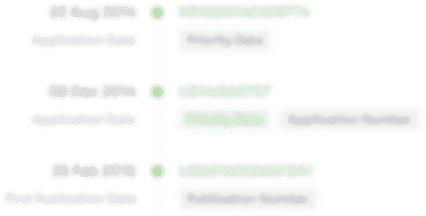
- R&D Engineer
- R&D Manager
- IP Professional
- Industry Leading Data Capabilities
- Powerful AI technology
- Patent DNA Extraction
Browse by: Latest US Patents, China's latest patents, Technical Efficacy Thesaurus, Application Domain, Technology Topic.
© 2024 PatSnap. All rights reserved.Legal|Privacy policy|Modern Slavery Act Transparency Statement|Sitemap