Convolutional-neural-network-based primary classification method for ear image of corn seed production
A convolutional neural network and corn ear technology, applied in the field of pattern recognition, can solve the problems of manpower and financial resources, slow screening speed, complex and changeable seed production site environment, etc.
- Summary
- Abstract
- Description
- Claims
- Application Information
AI Technical Summary
Problems solved by technology
Method used
Image
Examples
Embodiment Construction
[0031] The specific implementation manners of the present invention will be further described in detail below in conjunction with the accompanying drawings and embodiments. The following examples are used to illustrate the present invention, but are not intended to limit the scope of the present invention.
[0032] figure 1 It is a flowchart of a method for primary classification of ear images of corn seed production based on convolutional neural network in an embodiment of the present invention, as figure 1 As shown, the method includes:
[0033] S1. Obtain the original training sample set of corn ears and the corn ear test sample set. Both the original training sample set of corn ears and the test sample set of corn ears include two-dimensional color images of normal corn ears and two-dimensional images of corn ears with bracts. 2D color image and other 2D color images of corn ears;
[0034] S2, through the AlexNet first convolutional neural network, distinguish the type ...
PUM
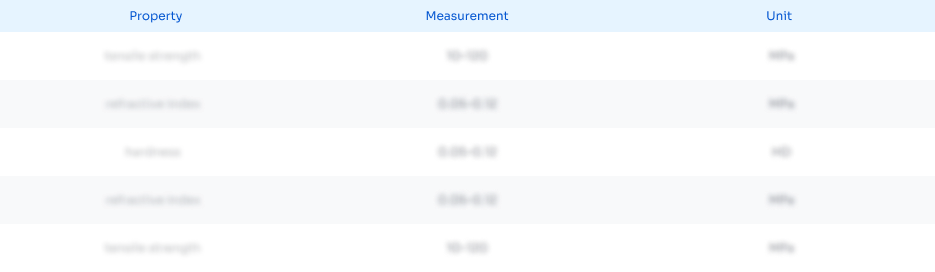
Abstract
Description
Claims
Application Information
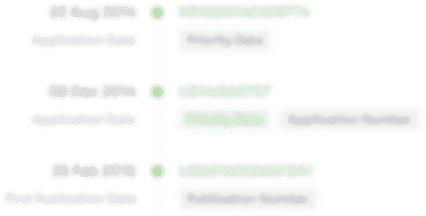
- R&D Engineer
- R&D Manager
- IP Professional
- Industry Leading Data Capabilities
- Powerful AI technology
- Patent DNA Extraction
Browse by: Latest US Patents, China's latest patents, Technical Efficacy Thesaurus, Application Domain, Technology Topic, Popular Technical Reports.
© 2024 PatSnap. All rights reserved.Legal|Privacy policy|Modern Slavery Act Transparency Statement|Sitemap|About US| Contact US: help@patsnap.com