Residual instance regression super-resolution reconstruction method based on multistage dictionary learning
A dictionary learning and super-resolution technology, applied in the field of image processing, can solve problems such as difficult to be widely used, difficult to express geometric structure information of low-resolution and high-resolution images, and high computational cost
- Summary
- Abstract
- Description
- Claims
- Application Information
AI Technical Summary
Problems solved by technology
Method used
Image
Examples
Embodiment Construction
[0046] In order to make the objects and advantages of the present invention clearer, the present invention will be further described in detail below in conjunction with the examples. It should be understood that the specific embodiments described here are only used to explain the present invention, not to limit the present invention.
[0047] refer to figure 1 with figure 2 , the embodiment of the present invention provides a residual instance regression super-resolution reconstruction method based on multi-level dictionary learning mainly includes two stages: a training set generation stage and an image super-resolution stage.
[0048] 1. Training set generation stage
[0049] Step 1: Simulate the image degradation process, build a feature library, and generate a training set.
[0050] 1a) Collect a large number of high-resolution gray-scale natural images, and generate corresponding low-resolution images for each high-resolution image through 4×4 average blurring and 3 t...
PUM
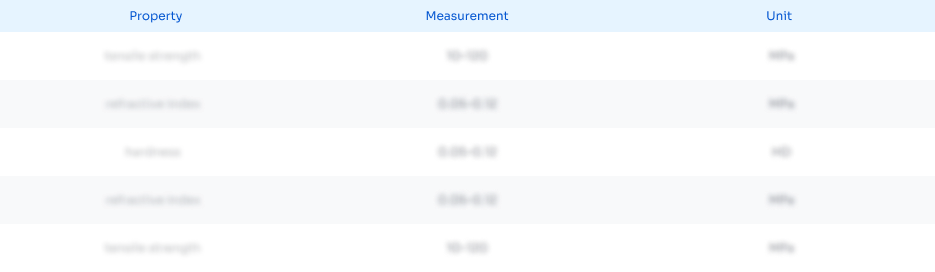
Abstract
Description
Claims
Application Information
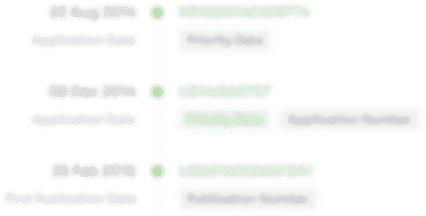
- R&D Engineer
- R&D Manager
- IP Professional
- Industry Leading Data Capabilities
- Powerful AI technology
- Patent DNA Extraction
Browse by: Latest US Patents, China's latest patents, Technical Efficacy Thesaurus, Application Domain, Technology Topic, Popular Technical Reports.
© 2024 PatSnap. All rights reserved.Legal|Privacy policy|Modern Slavery Act Transparency Statement|Sitemap|About US| Contact US: help@patsnap.com