Epilepsy seizure prediction method based on semi-supervised deep generative adversarial network
A prediction method and epileptic seizure technology, applied in the field of EEG signal processing technology and deep learning, can solve the problem of difficulty in manual screening of features, and achieve the effect of optimizing performance and high classification accuracy.
- Summary
- Abstract
- Description
- Claims
- Application Information
AI Technical Summary
Problems solved by technology
Method used
Image
Examples
Embodiment Construction
[0025] The present invention will be further described below in conjunction with the accompanying drawings and specific embodiments.
[0026] Step one, data acquisition.
[0027] The method of the present invention is applied to the intracranial EEG data of five groups of canine epilepsy subjects in the intracranial EEG data jointly developed by the University of Pennsylvania and the Mayo Clinic. Using intracranial EEG to locate epileptic foci in the brain to facilitate surgery and prevent future seizures. The data set was collected using different numbers of electrodes, the sampling frequency was 400 Hz, and the reference electrodes were extracranial electrodes. The data is divided into segments every ten minutes, and the data categories are divided into preictal and interictal. The data segments are numbered in chronological order, and the test data are randomly arranged. The preictal period included data for a total of one hour's duration five minutes before the onset (1...
PUM
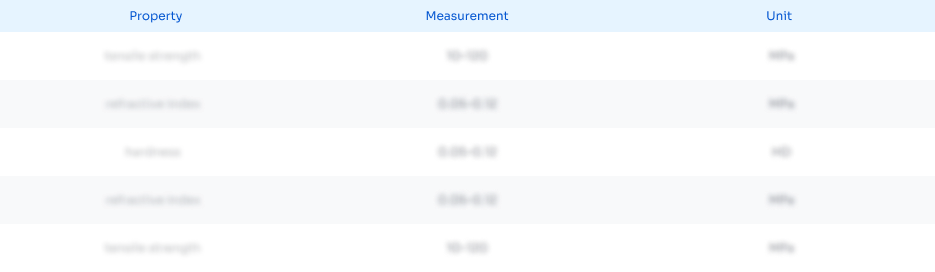
Abstract
Description
Claims
Application Information
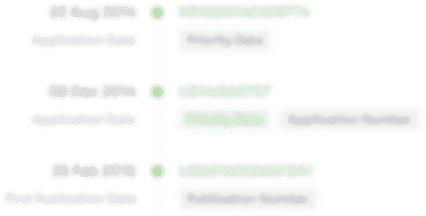
- R&D
- Intellectual Property
- Life Sciences
- Materials
- Tech Scout
- Unparalleled Data Quality
- Higher Quality Content
- 60% Fewer Hallucinations
Browse by: Latest US Patents, China's latest patents, Technical Efficacy Thesaurus, Application Domain, Technology Topic, Popular Technical Reports.
© 2025 PatSnap. All rights reserved.Legal|Privacy policy|Modern Slavery Act Transparency Statement|Sitemap|About US| Contact US: help@patsnap.com