Digital image denoising method based on NSST and CNN
A technology of digital image and noise reduction, applied in the field of digital image noise reduction based on NSST and CNN, can solve the problems of loss of image details and excessive blurring, to make up for excessive blurring of images, make up for loss of image details and information, and protect image edges. The effect of detailed information
- Summary
- Abstract
- Description
- Claims
- Application Information
AI Technical Summary
Problems solved by technology
Method used
Image
Examples
Embodiment Construction
[0040] like figure 1 Shown, the digital image denoising method of the present invention based on NSST and CNN comprises the steps:
[0041] Step 1: Network training phase, the specific steps are as follows:
[0042] Step 1.1: Carry out Ascombe transformation by Ascombe transformation module 101, convert the noise that approximately obeys the Poisson distribution in the image into the noise that approximately obeys the standard Gaussian distribution;
[0043] Step 1.2: Perform NSST transformation (non-subsampling shearlet transformation) through the NSST transformation module 102, decompose the noise image and its corresponding high-quality image into multi-level sub-band images through the data set production module, and divide each level of sub-band The images with images are respectively cut into image blocks of a certain size as a data set;
[0044] Step 1.3: Based on the data set obtained in step 1.2, CNN training is performed through the network model training module fo...
PUM
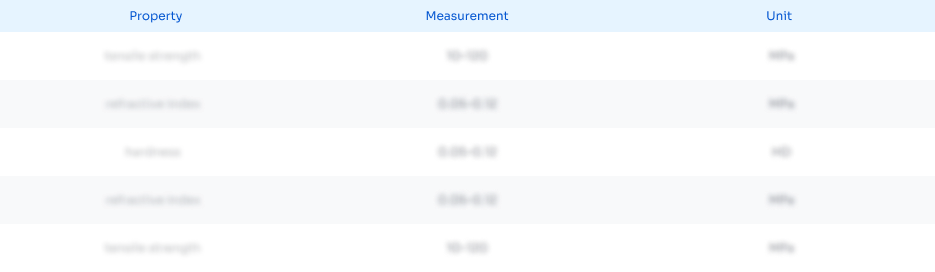
Abstract
Description
Claims
Application Information
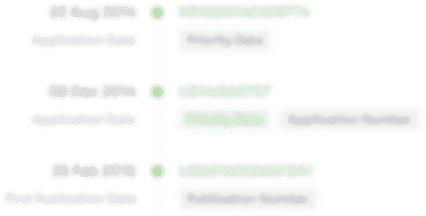
- R&D Engineer
- R&D Manager
- IP Professional
- Industry Leading Data Capabilities
- Powerful AI technology
- Patent DNA Extraction
Browse by: Latest US Patents, China's latest patents, Technical Efficacy Thesaurus, Application Domain, Technology Topic, Popular Technical Reports.
© 2024 PatSnap. All rights reserved.Legal|Privacy policy|Modern Slavery Act Transparency Statement|Sitemap|About US| Contact US: help@patsnap.com