Solar cell broken gate defect detection method based on convolutional neural network
A convolutional neural network, solar cell technology, applied in image data processing, instrument, character and pattern recognition, etc., can solve the problem of high false alarm rate, achieve the effect of improving complexity and solving the lack of data volume
- Summary
- Abstract
- Description
- Claims
- Application Information
AI Technical Summary
Problems solved by technology
Method used
Image
Examples
Embodiment Construction
[0037] The preferred embodiments of the present invention will be described in detail below with reference to the accompanying drawings.
[0038] The present invention provides a method for detecting defects in solar cell fragment grids based on convolutional neural networks, such as figure 1 ,include:
[0039] S1. Clean the collected solar cell pictures and divide them into training set and test set;
[0040] S2. Using the horizontal integral projection method to extract the regions of interest RIO of the training set and the test set respectively, and then divide the regions of interest of the training set and the test set into several image blocks;
[0041] S3. According to the number of defective pixels contained in the central area of the image block, divide the image blocks of the training set into positive samples and negative samples, and perform data enhancement on the positive samples and randomly sample the negative samples;
[0042] S4. Input the positive sampl...
PUM
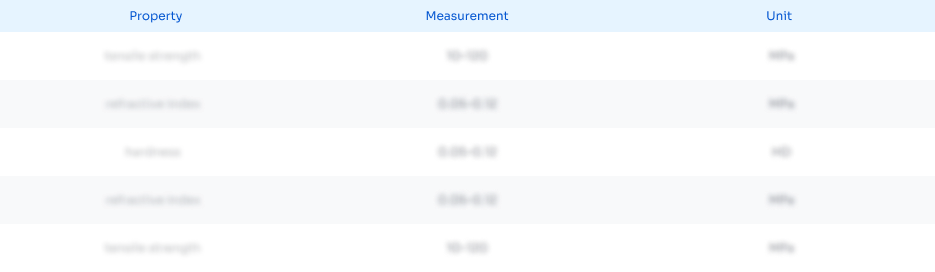
Abstract
Description
Claims
Application Information
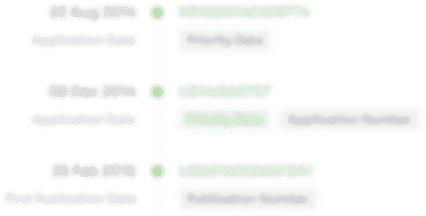
- R&D Engineer
- R&D Manager
- IP Professional
- Industry Leading Data Capabilities
- Powerful AI technology
- Patent DNA Extraction
Browse by: Latest US Patents, China's latest patents, Technical Efficacy Thesaurus, Application Domain, Technology Topic, Popular Technical Reports.
© 2024 PatSnap. All rights reserved.Legal|Privacy policy|Modern Slavery Act Transparency Statement|Sitemap|About US| Contact US: help@patsnap.com