Improved deep convolutional neural network-based remote sensing image classification model
A technology of remote sensing images and neural networks, applied in the field of remote sensing images, can solve the problems of no corresponding disclosure, etc., achieve the effects of reducing the generation of model parameters, high computing efficiency, and reducing computing resource consumption
- Summary
- Abstract
- Description
- Claims
- Application Information
AI Technical Summary
Problems solved by technology
Method used
Image
Examples
Embodiment Construction
[0071] The specific embodiments provided by the present invention will be described in detail below in conjunction with the accompanying drawings.
[0072] A remote sensing image classification model based on an improved deep convolutional neural network. The remote sensing image classification model is mainly reflected in the following two aspects: (1) Aiming at the multi-spectral characteristics of remote sensing images, the structure of the deep convolutional neural network is optimized. The bottleneck unit is set in the convolution structure of the deep convolutional neural network to realize the dimensionality reduction of the input remote sensing image; through group convolution, the convolution calculation amount during the training of the deep convolutional neural network remote sensing image classification model is reduced; at the same time, for Spatial correlation of remote sensing images, building a channel shuffling structure, and improving the feature extraction ab...
PUM
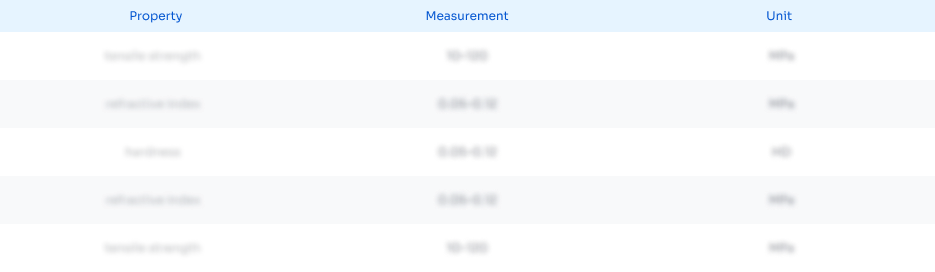
Abstract
Description
Claims
Application Information
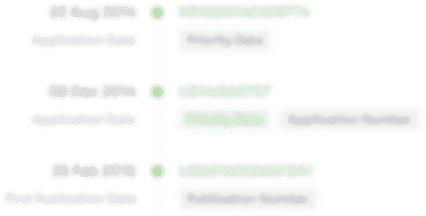
- R&D
- Intellectual Property
- Life Sciences
- Materials
- Tech Scout
- Unparalleled Data Quality
- Higher Quality Content
- 60% Fewer Hallucinations
Browse by: Latest US Patents, China's latest patents, Technical Efficacy Thesaurus, Application Domain, Technology Topic, Popular Technical Reports.
© 2025 PatSnap. All rights reserved.Legal|Privacy policy|Modern Slavery Act Transparency Statement|Sitemap|About US| Contact US: help@patsnap.com