Face image recovery method and device based on cyclic neural network
A technology of cyclic neural network and face image, applied in biological neural network model, neural architecture, image enhancement, etc., can solve the problems of increasing the number of input image samples, unable to improve the reconstruction effect, and low recovery rate
- Summary
- Abstract
- Description
- Claims
- Application Information
AI Technical Summary
Problems solved by technology
Method used
Image
Examples
no. 1 example
[0055] See figure 1 , figure 1 It is a schematic flowchart of a face image restoration method based on a recurrent neural network provided by the first embodiment of the present invention.
[0056] The face image restoration method based on cyclic neural network includes the following steps:
[0057] S101. Obtain a low-resolution image, and perform face detection on the low-resolution image to obtain a face image to be restored.
[0058] Specifically, a low-resolution image is obtained; a template matching algorithm is used to calculate the mean and variance of the low-resolution image area; when the pre-built face template is used to match the low-resolution image area, the person is calculated The correlation coefficient between the face template and the low-resolution image area; the correlation coefficient is compared with a preset threshold to determine the face area, and the face image to be restored containing at least one face is filtered out.
[0059] In this embodiment, the ...
no. 2 example
[0104] See Figure 5 , Figure 5 It is a schematic structural diagram of a face image restoration device based on cyclic neural network provided by the second embodiment of the present invention.
[0105] The face image restoration device based on cyclic neural network includes:
[0106] The face image acquiring unit 301 to be restored is configured to acquire a low-resolution image and perform face detection on the low-resolution image to obtain a face image to be restored.
[0107] In this embodiment, the method for constructing the face template is:
[0108] Collecting face samples, and converting the face samples into grayscale images; the face samples include face images, gender, age, and race;
[0109] Intercepting the face area including eyes, nose, mouth, chin and eyebrows in the face image;
[0110] Normalize the size of the face area to meet the requirements of the aspect ratio and area size of the face area, and obtain a preliminary face template;
[0111] Calculate the mean an...
PUM
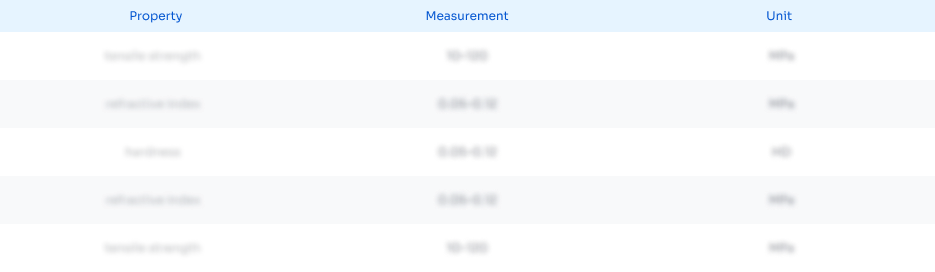
Abstract
Description
Claims
Application Information
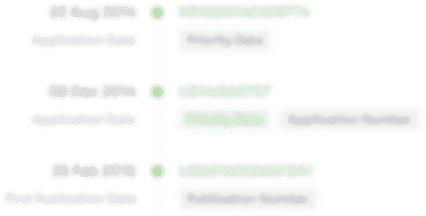
- R&D
- Intellectual Property
- Life Sciences
- Materials
- Tech Scout
- Unparalleled Data Quality
- Higher Quality Content
- 60% Fewer Hallucinations
Browse by: Latest US Patents, China's latest patents, Technical Efficacy Thesaurus, Application Domain, Technology Topic, Popular Technical Reports.
© 2025 PatSnap. All rights reserved.Legal|Privacy policy|Modern Slavery Act Transparency Statement|Sitemap|About US| Contact US: help@patsnap.com