Training method for classification model, and device and computer server thereof
A classification model and classifier technology, applied in the field of deep learning, can solve the problems of narrow application scope and low calculation efficiency of classification models, and achieve the effect of wide application range and improved training efficiency.
- Summary
- Abstract
- Description
- Claims
- Application Information
AI Technical Summary
Problems solved by technology
Method used
Image
Examples
Embodiment 1
[0035] see figure 1 , which is a flowchart of a training method for a classification model in an embodiment of the present invention, the method includes:
[0036] Step 101, construct an initial classification model, the initial classification model includes at least one single-modal classification model with the same classification task, wherein the modal data training set corresponding to each single-modal classification model includes labeled training data and unlabeled training data data;
[0037] Step 102, based on the method of aligning the feature encoding distribution of the labeled training data and the unlabeled training data in the modal data training set of each unimodal classification model, using the modal training data set pair corresponding to each unimodal classification model The initial classification model is trained to obtain a target classification model.
[0038] In the embodiment of the present invention, each unimodal classification model in the init...
example 1
[0041] In Example 1, the structure of the initial classification model can be as follows figure 2 Shown contains only one unimodal classification model, it can also be shown as image 3 shown contains more than two unimodal classification models, whether figure 2 still image 3 As shown in the structure, each unimodal classification model includes a feature encoder and a classifier and a discriminator respectively cascaded with the feature encoder, and the discriminator is used to judge that the feature encoding output by the feature encoder comes from Labeled training data or unlabeled training data, the output of the discriminator is provided with a first loss function for training the discriminator and a second loss function for training the feature encoder, the The first loss function and the second loss function adversarial settings.
[0042] In this example 1, the step 102 uses the modal training data sets corresponding to each unimodal classification model to train...
example 2
[0054] The structure of setting up the initial classification model can be as follows Figure 7 As shown, each unimodal classification model includes a feature encoder and a classifier and a discriminator cascaded with the feature encoder respectively, and the discriminator is used to judge that the feature encoding output by the feature encoder comes from a labeled Training data or unlabeled training data, the output of the discriminator is provided with a first loss function for training the discriminator and a second loss function for training the feature encoder, the first The loss function and the second loss function confrontation setting; and the feature encoders of multiple unimodal classification models are also respectively connected to the same cross-modal discriminator, and the cross-modal discriminator is used to distinguish each unimodal classification model The modal type corresponding to the feature encoding output by the feature encoder of the feature encoder,...
PUM
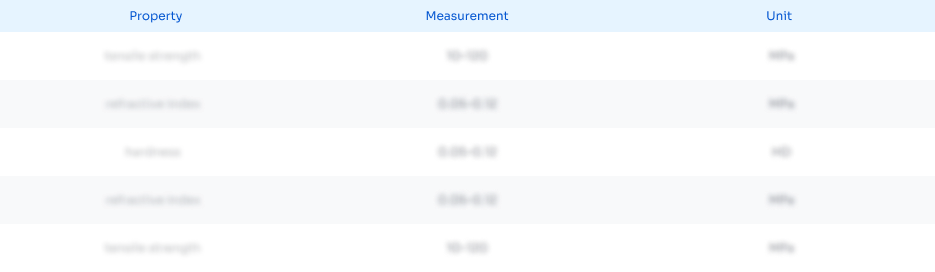
Abstract
Description
Claims
Application Information
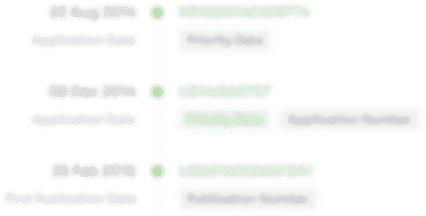
- R&D
- Intellectual Property
- Life Sciences
- Materials
- Tech Scout
- Unparalleled Data Quality
- Higher Quality Content
- 60% Fewer Hallucinations
Browse by: Latest US Patents, China's latest patents, Technical Efficacy Thesaurus, Application Domain, Technology Topic, Popular Technical Reports.
© 2025 PatSnap. All rights reserved.Legal|Privacy policy|Modern Slavery Act Transparency Statement|Sitemap|About US| Contact US: help@patsnap.com