Deep convolutional neural network based fine grain electrocardiogram signal classification method fusing with online decisions
A technique of electrocardiogram and deep convolution, applied in the field of signal classification, to achieve the effect of high computational efficiency
- Summary
- Abstract
- Description
- Claims
- Application Information
AI Technical Summary
Problems solved by technology
Method used
Image
Examples
Embodiment Construction
[0035] The present invention will be further described below in conjunction with accompanying drawing.
[0036] Such as figure 1 As shown, the present invention proposes fine-grained ECG signal classification based on deep convolutional neural network and online decision fusion, including the following steps:
[0037] Step (1) Acquisition and processing of ECG signal waveform:
[0038] Step (1-1) Data set acquisition: SKX-2000 ECG signal simulator was used for ECG waveform generation. The simulator is capable of simulating ECG waveforms with various amplitudes and frequencies of different symptoms, including but not limited to more than 20 types of ECG waveforms such as normal, coarse atrial fibrillation, fine atrial fibrillation, and atrial flutter. For the above 20 types of ECG waveform signals, a certain amount of waveform signals under different parameters corresponding to the categories are respectively collected. Mathematically expressed as:
[0039] X={(x i (t),y ...
PUM
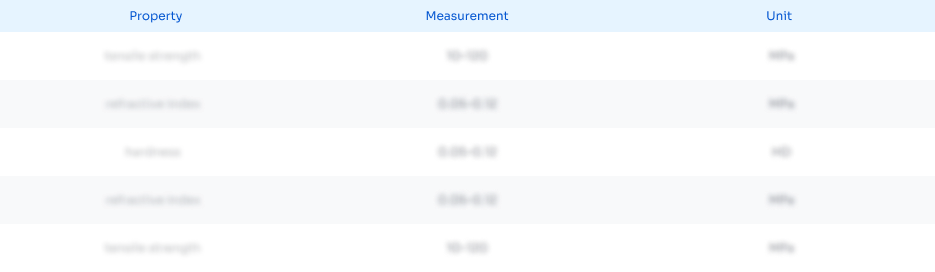
Abstract
Description
Claims
Application Information
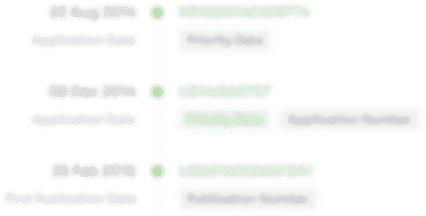
- R&D Engineer
- R&D Manager
- IP Professional
- Industry Leading Data Capabilities
- Powerful AI technology
- Patent DNA Extraction
Browse by: Latest US Patents, China's latest patents, Technical Efficacy Thesaurus, Application Domain, Technology Topic, Popular Technical Reports.
© 2024 PatSnap. All rights reserved.Legal|Privacy policy|Modern Slavery Act Transparency Statement|Sitemap|About US| Contact US: help@patsnap.com