Deep Q neural network anti-interference model and intelligent anti-interference algorithm
A neural network and model technology, applied in biological neural network models, neural learning methods, neural architectures, etc., can solve problems such as the inability to expand the application environment of state decision space, and achieve the effect of reducing computational complexity
- Summary
- Abstract
- Description
- Claims
- Application Information
AI Technical Summary
Problems solved by technology
Method used
Image
Examples
Embodiment 1
[0101] The first embodiment of the present invention is specifically described as follows. The system simulation adopts the python language, based on the caffe deep learning framework, and the parameter setting does not affect the generality. This example verifies the effectiveness of the proposed model and method, Figure 4 Validation of effectiveness against fixed jamming patterns. The parameters are set as follows: the interference and user frequency bands are 20MHz, and the frequency resolution of spectrum sensing is 100kHz. Users perform full-band sensing every 1ms and keep the perceived spectrum data for 200ms. Therefore, S t The matrix size is 200×200, the user signal bandwidth is 4MHz, and the user center frequency changes by 2MHz every 10ms, so K=9. Both the signal waveforms of the user and the interference are raised cosine waves, and the roll-off coefficient is α=0.5. The interference power is 30dBm, and the user's signal power is 0dBm. Demodulation Threshold β ...
Embodiment 2
[0105] The second embodiment of the present invention is specifically described as follows. The system simulation adopts the python language, based on the caffe deep learning framework, and the parameter setting does not affect the generality. This example verifies the effectiveness of the proposed model and method, Figure 4 Verify the effectiveness against fixed jamming patterns, Figure 5 Verify the effectiveness of countering dynamic jamming and intelligent jamming. The parameters are set as follows: the interference and user frequency bands are 20MHz, and the frequency resolution of spectrum sensing is 100kHz. Users perform full-band sensing every 1ms and keep the perceived spectrum data for 200ms. Therefore, S t The matrix size is 200×200, the user signal bandwidth is 4MHz, and the user center frequency changes by 2MHz every 10ms, so K=9. Both the signal waveforms of the user and the interference are raised cosine waves, and the roll-off coefficient is α=0.5. The inte...
PUM
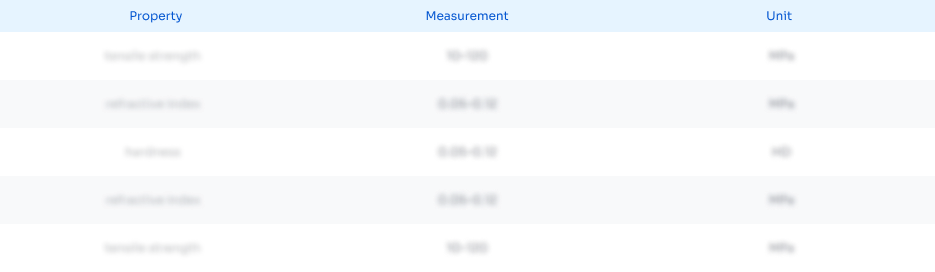
Abstract
Description
Claims
Application Information
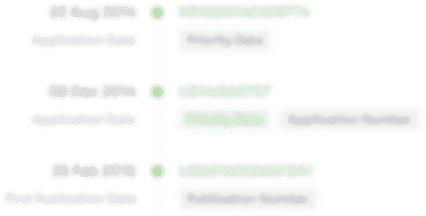
- R&D
- Intellectual Property
- Life Sciences
- Materials
- Tech Scout
- Unparalleled Data Quality
- Higher Quality Content
- 60% Fewer Hallucinations
Browse by: Latest US Patents, China's latest patents, Technical Efficacy Thesaurus, Application Domain, Technology Topic, Popular Technical Reports.
© 2025 PatSnap. All rights reserved.Legal|Privacy policy|Modern Slavery Act Transparency Statement|Sitemap|About US| Contact US: help@patsnap.com