Just-in-time learning soft measurement modeling method based on Bayes Gaussian mixture model
A hybrid model and modeling method technology, applied in character and pattern recognition, special data processing applications, instruments, etc., can solve the problem of inability to fully consider the non-Gaussian nature of process data, and achieve the effect of reducing production costs and improving product quality.
- Summary
- Abstract
- Description
- Claims
- Application Information
AI Technical Summary
Problems solved by technology
Method used
Image
Examples
Embodiment
[0056] This embodiment provides a real-time learning soft sensor modeling method based on the Bayesian Gaussian mixture model. This embodiment takes a common chemical process——butanizer process as an example. The experimental data comes from the debutanizer process to predict the butane concentration, see figure 1 , the method includes:
[0057] Step 1: Collect input and output data to obtain a historical training data set.
[0058] Step 2: Given the training sample X, use BIC to determine the optimal number K of Gaussian components. The description of BIC is as formula (1):
[0059] BIC=-2logp(X|Θ)+dlog(N) (1)
[0060] In formula (1), logp(X|Θ) represents the logarithmic likelihood function of the training samples, d represents the number of free parameters of the K Gaussian components, and N represents the number of training samples
[0061] Step 3: After obtaining the optimal number K of Gaussian components, given the initial parameters of the Gaussian mixture model (GM...
PUM
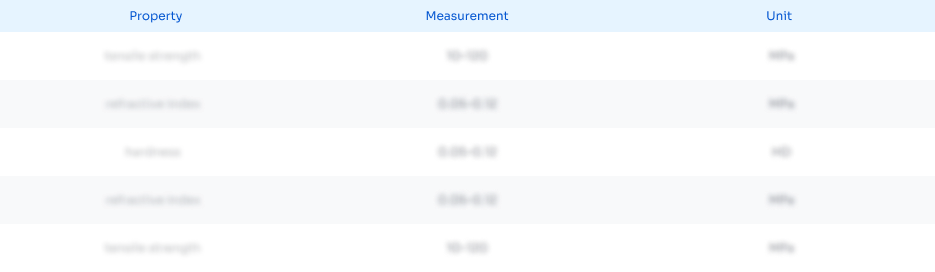
Abstract
Description
Claims
Application Information
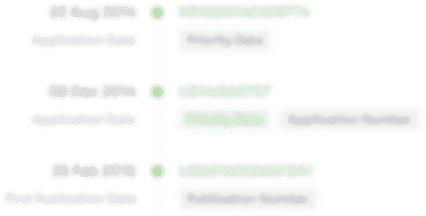
- R&D
- Intellectual Property
- Life Sciences
- Materials
- Tech Scout
- Unparalleled Data Quality
- Higher Quality Content
- 60% Fewer Hallucinations
Browse by: Latest US Patents, China's latest patents, Technical Efficacy Thesaurus, Application Domain, Technology Topic, Popular Technical Reports.
© 2025 PatSnap. All rights reserved.Legal|Privacy policy|Modern Slavery Act Transparency Statement|Sitemap|About US| Contact US: help@patsnap.com