Abnormal electricity utilization user detection method based on semi-supervised learning
A semi-supervised learning and abnormal power consumption technology, applied in the field of detection, can solve the problem of model training without a training set, and achieve the effect of improving accuracy and efficiency
- Summary
- Abstract
- Description
- Claims
- Application Information
AI Technical Summary
Problems solved by technology
Method used
Image
Examples
Embodiment Construction
[0040] Below in conjunction with accompanying drawing and specific embodiment the present invention is described in further detail:
[0041] 1. Model steps and framework
[0042] The inventive method realization work mainly comprises the following steps:
[0043] First, assuming that most people are normal users, and the behavior characteristics of normal users and abnormal (stealing power) users are different, use the cluster analysis method to screen out outlier users, that is, get the first-level gray list.
[0044] Secondly, based on the first-level gray list, calculate the outlier degree (LOF value) of the user, judge the user's suspicious degree according to the outlier degree, and form a second-level gray list with suspicious degree ranking.
[0045] The third step, based on the secondary gray list, go to the scene to collect fraudulent evidence of outlier users, obtain a blacklist, and store it in the blacklist database.
[0046] In the fourth step, in view of the fa...
PUM
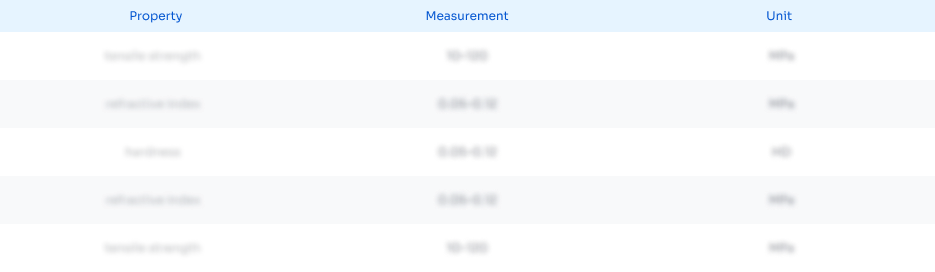
Abstract
Description
Claims
Application Information
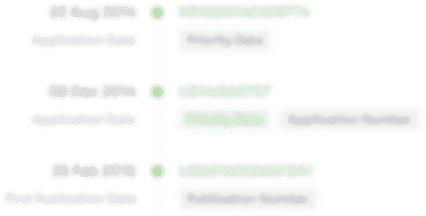
- R&D Engineer
- R&D Manager
- IP Professional
- Industry Leading Data Capabilities
- Powerful AI technology
- Patent DNA Extraction
Browse by: Latest US Patents, China's latest patents, Technical Efficacy Thesaurus, Application Domain, Technology Topic, Popular Technical Reports.
© 2024 PatSnap. All rights reserved.Legal|Privacy policy|Modern Slavery Act Transparency Statement|Sitemap|About US| Contact US: help@patsnap.com