Zero sample image classification method based on combination of variational autocoder and adversarial network
A technology of sample images and classification methods, used in computer parts, neural learning methods, biological neural network models, etc.
- Summary
- Abstract
- Description
- Claims
- Application Information
AI Technical Summary
Problems solved by technology
Method used
Image
Examples
Embodiment Construction
[0055] The present invention provides a zero-sample image classification method based on the combination of variational autoencoder and confrontation network, which is guided by the sample category mapping in the training set (category mapping in this method refers to the attribute label of each category) . When training the model, the samples of known categories and the attribute labels corresponding to the samples are used as the input of the model, and the parameters of the network are backpropagated through five loss functions: reconstruction loss, generation loss, discrimination loss, divergence loss, and classification loss. After the model training is completed, the random Gaussian noise of the input sample and the attributes of the unknown category are generated to generate the corresponding pseudo samples of the unknown category, and then the pseudo samples are used to train the classifier to test on the samples of the unknown category.
[0056] see figure 1, the pre...
PUM
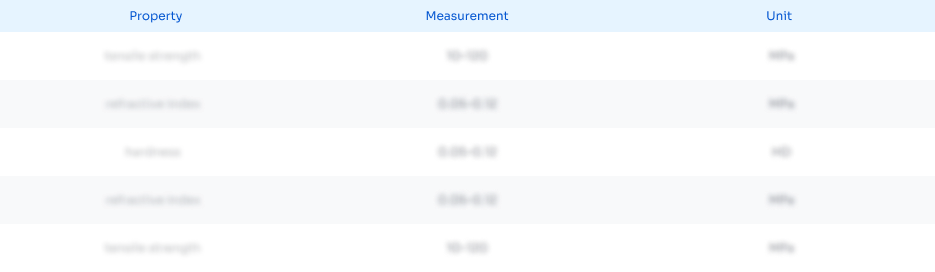
Abstract
Description
Claims
Application Information
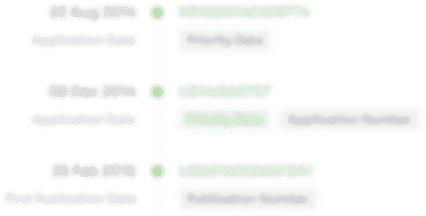
- R&D Engineer
- R&D Manager
- IP Professional
- Industry Leading Data Capabilities
- Powerful AI technology
- Patent DNA Extraction
Browse by: Latest US Patents, China's latest patents, Technical Efficacy Thesaurus, Application Domain, Technology Topic, Popular Technical Reports.
© 2024 PatSnap. All rights reserved.Legal|Privacy policy|Modern Slavery Act Transparency Statement|Sitemap|About US| Contact US: help@patsnap.com